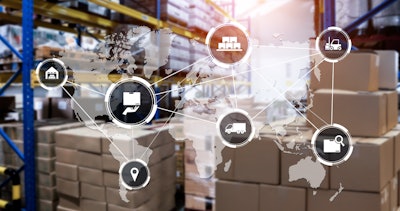
Today’s supply chain executives face a multitude of challenges, from climate change to the ongoing labor shortage. Amid growing regulatory and financial pressure to reduce energy consumption and emissions and achieve high-level sustainability goals, demographic changes have created increased pressure to run plants more efficiently with fewer personnel.
In this complex production environment, digital twins can be highly effective tools for optimizing supply chain processes without wasting time and resources. This article will examine three interesting use cases where digital twins can improve manufacturing operations: increasing sustainability, dealing with the labor shortage, and creating a circular economy.
Before beginning, it’s necessary to briefly define the phrase “digital twin.” In the broadest of terms, anything can be a digital twin, even an Excel chart. Therefore, for the purposes of this article, let’s define a digital twin as: A virtual copy of a process that makes your facility more efficient.
How digital twins increase sustainability
Making supply chains more sustainable can be a challenge, because the work is so siloed. Typically you will see different teams working on different objectives. Some are optimizing quality, while others are trying to cut costs. As a result, sustainability often ends up falling into the bucket of lower-tier priorities.
But digital twins change the game. Using historical data, a digital twin can be built that creates a virtual copy of an existing factory. Engineers can then use this tool in conjunction with machine learning models to create a more efficient supply chain that optimizes for every goal at the same time, from cutting costs to reducing greenhouse gas emissions.
Click here to hear more about digital twins:
How digital twins deal with the labor shortage
As seasoned engineers and operators retire from positions across the supply chain, it is crucial to train less-experienced workers to replace them. A digital twin can be a quick and inexpensive training tool.
How does this work in practice? The digital twin captures the experience of existing operators and engineers through historical data, then uses what has worked in the past to make recommendations for less-experienced hires. Once new trainees feel confident in their decision-making skills, they can use the digital twin to test them out, without affecting physical operations.
One challenge in this regard is making sure the digital twin is an effective teaching tool. It is not effective if the software is just telling you what to do, and participants are mindlessly doing whatever it tells them. A digital twin that tells users why certain outcomes occur is necessary in order to foster an educational experience for recipients, which is vital for ensuring they go on to become participants in the digitalized workforce.
How digital twins help create a circular economy
The theory behind a circular economy is that the waste of one plant can become the input of another, hence eliminating waste as we know it. But one major challenge preventing this from becoming a reality is variability in raw materials. Waste does not always look the same. For example, one chemical producer might discard a substance with a particular composition which another plant might not be accustomed to using in production.
Digital twins can help plants adapt to such real-life variability. Engineers at the receiving end can use digital twins to figure out how to use what they have been given in the most efficient way, without having to resort to physical testing. Without digital twins, perhaps there would be no circular economy.
Click here to hear more about the circular economy:
The challenges
Incorporating digital twins, as with any project in the arena of digitalization, is not without its challenges. Data connectivity, in particular, can be a significant and complex hurdle for manufacturers and supply chain executives. How do you get data from one plant to another? Or within a single plant? Even in one factory, not all parts are connected. Sharing data across plants can be an even more difficult and daunting problem.
Data connectivity is improving slowly, as the industrial sector becomes more standardized regarding the data platforms it uses. The implementation of similar databases with similar interfaces across factories makes it far easier to connect and exchange data across digital twins than it once was. However, the industrial world is not always as high-tech as one might imagine, and infrastructural challenges remain, preventing the implementation of digital twins that could make production more efficient.
Data cleanliness is yet another challenge limiting the adoption of digital twins and their capability to drive efficiency across the supply chain. For a digital twin to drive value, the underlying software needs to have a supply of high-quality data. An increasing number of supply chain executives are tapping into machine learning solutions, as these software tools can not only help build a digital twin, but also clean the data before it goes into the software. If there are a lot of errors in your data, a digital twin will provide little value