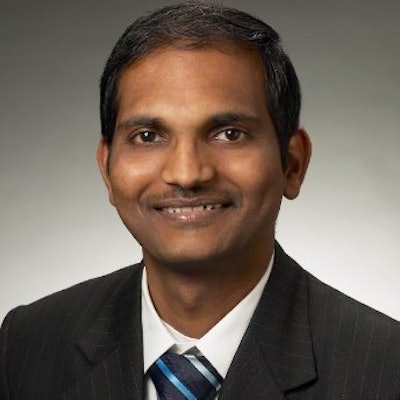
For many years, we have been successfully utilizing inventory management concepts such as safety stock, economic order quantity, cost of goods, inventory turnover and vendor-managed inventory, among others. As the title suggests, our discussion is focused toward managing maintenance, repair and overhaul (MRO) spare parts inventory. It is well understood that businesses have to maintain safety stock spare parts to support the unexpected breakdown of equipment, and it is fair to say that we need to find a balance between non-stock and stock items, and their minimum and maximum quantity.
What is reliability? Reliability is theoretically defined as the probability of success (Reliability=1-Probability of Failure). Reliability engineering deals with the estimation, prevention and management of high levels of lifetime engineering uncertainty and risk of failure.
Anything that can be measured follows some kind of distribution model, be it widely used normal distribution, or Poisson or gamma distribution. If the consumption pattern is fairly regular at a certain frequency and the quantity is greater than 300 per year, which is almost one unit per day in a year, this event can be classified as normal distribution to calculate the safety stock. However, MRO spares are seldom consumed in hundreds of units. In general, 30 to 40 percent of the items are not consumed in a given year, 50 to 60 percent of the items are consumed between 1 and 299 in a year or less than one unit per day in a given year, and only 5 to 10 percent of the items are consumed at greater than 300 in a year or one unit per day.
I am going to label the scenarios as:
Consumption in a Given Year |
Label |
0 |
Very Low Consumption |
1–299 |
Low Consumption |
300 and more |
Mass Consumption |
Most of the concepts that are widely used today operate under a huge assumption that the consumption is at a fairly regular frequency and reasonable quantity, or in other words, follows normal distribution. Before we go in-depth, I want to highlight that the above classification is for spare parts with a consumption history. So how do we determine the safety stock for new spare parts with no consumption history? This is another scenario that needs to be addressed. New spare parts are typically spares recommended by the original equipment manufacturer (OEM) when you buy new machinery.
I will use this post to explain the skeleton model and, in a later post, I will explain the various mathematical models in detail.
Very Low Consumption Items
Maintain zero or one unit of stock by determining the item criticality level—vital, essential and desirable (VED) based on factors such as functionality, response time, lead time, commodity nature of the item, lifecycle stage, demand and price. Taking these factors into account, perform an analytic hierarchy methodology (AHM) and arrive at a quantitative score to determine the VED characteristic of the target item. Based on the VED label, maintain one unit in stock for vital items, and maintain no stock for essential and desirable items.
Low Consumption Items
Consider a three-year (at least one year if data is not available) consumption pattern to determine the safety stock of the items. Based on the history, identify the input variables for the model such as daily mean, daily variance, maximum unit consumed in one instance and lead time. If the daily variance and the daily mean consumption of the item is within 10 percent, use Poisson distribution to determine the safety stock.
Poisson distribution is given by:
X is the consumption of spare parts per time interval whose probability is to be estimated, t is the time interval to be considered, ƛ is the historical rate of consumption per unit of time, and Px(t) is the probability of occurring x requests during time interval t.
If the daily variance and the daily mean consumption of the item is greater than 10 percent, use the bootstrapping technique to understand the frequency and probability of consumption during the lead time of the item to maintain the target service level.
Mass Consumption Items
Determine the safety stock by using the standard normal distribution formula.
New Spare Parts or Items with No History
Components typically exhibit the reliability bathtub curve behavior, which is a combination of a decreasing hazard of early failure, and an increasing hazard of wear-out failure, and a constant hazard of random failure.
A certain number of failures occur over a certain length of time for equipment exhibiting a constant failure rate. They are Poisson distributed, occur at a constant average rate and the number of events occurring in any time interval is independent of the number of events occurring in any other time interval.
I reckon several not-so-common terminologies, such as AHM and bootstrapping, among others, were introduced in this post and I shall talk about these in detail, while I explain the model and methodologies in my next post.