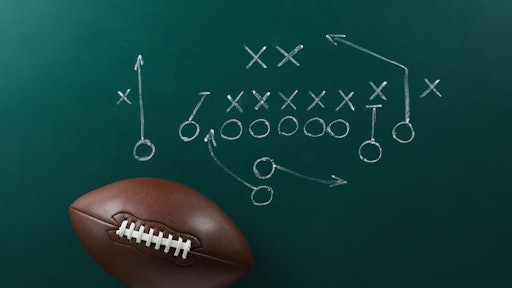
At the time of writing this, the Buffalo Bills, based on thousands of simulations, were projected to win the Super Bowl (please let my past self-know if he should go buy a lottery ticket!). The use of analytics in sports has been around for decades, becoming even more prevalent in recent years with the evolution of more powerful technology and the emergence of Artificial Intelligence (AI). Incidentally, the use of analytics in sports can serve as a strong reference for how you can better configure your supply chain to identify potential risks and analyze past errors or wins to find hidden relationships that will help you outperform your competitors.
In the early 2000s, football teams were using spreadsheets and statistical software to analyze data and gain a deeper understanding of player performance and strategies. Fast-forward to the present– AI and machine learning now allow teams to analyze large amounts of data quickly and more accurately, enabling analysts to make data-driven predictions about player performance and game outcomes.
As part of this the type and level of granularity of the detail used has evolved. The NFL (National Football League), for example, has moved from basic metrics like passing yards and rushing yards, into advanced analytics based on the explosion of contextual data– setting a fine example for companies looking to make the most of data and analytics to drive improvements in the supply chain and ultimately make more informed decisions.
How Pro Football is Harnessing AI and ML
The football world has seen a mind-boggling increase in the types of data from Next Gen Stats, Pro Football Focus and Football Outsiders, that are leveraged by analysts to provide insights into why teams win and how well players perform, as well as the underlying schemes, decisions or skills behind these discrete events.
NFL’s Next Gen Stats (NGS) tracks and codifies player qualitative data like the fastest ‘get off’ for pass rushers, the ‘largest separation’ for receivers and more, for individual players. This data allows for tremendous insights about the relative upside, or risk, of players in comparison to their peers, and can be used to contextualize performance based on more thorough analysis. NGS also provides contextualized data on how a player performs relative to how their peers would perform in similar circumstances, which includes factors like Completion Percentage Over Expected (CPOE), Rush Yards Over Expected, and Expected Sacks.
OK, great. But where does AI/ML come in? The role of AI/ML is in the how and why players are projected to be leaders, or stumble, as the season progresses. An example is Haason Reddick, a player who is now considered a ‘Free Agent Steal’ after accruing 12.5 sacks before signing ahead of the 2022 season with the Carolina Panthers. In 2021 he had 11 sacks, only to explode this last season with 16 sacks. Analysis by ESPN and The Liberty Line saw this coming thanks to contextual insights in the data. What is compelling about this analysis is that it derived from the possibility of Reddick joining a different team, the Philadelphia Eagles, and playing alongside Javon Hargrave and Fletcher Cox. The Liberty Line analysis contextualized the impact of having Hargrave, who was double-teamed at a rate 60% higher than the average Defensive Tackle, alongside Haason Reddick. While ESPN projected 9.5 sacks based on pass rush opportunity and win-rate (he ended with 16), the inclusion of the impact of having a feared pass rusher next to Reddick made The Liberty Line project even greater heights.
Machine Learning and The Power of Simulations
Machine Learning, a subset of AI, is proving remarkably valuable in sports to analyze performance and past outcomes to learn from what worked and what didn’t. It’s even more powerful when the insights are combined with data that is available but hasn’t yet been used in projections.
For instance, last September, much of the NFL was celebrating the impact of a series of high-profile wide receiver trades. Ahead of the season, Cynthia Frelund, a sports analyst, ran more than one million simulations of the 2022 season to predict the playoff field. She projected the Las Vegas Raiders would miss the playoffs. Rather than focusing on the addition of Davante Adams at Wide Receiver when analyzing the results of the one million simulations, Frelund found that the Raiders were destined to fail due to their ‘brutal schedule’.
This type of learning is what AI/ML brings to analysis; to not just project the future, but to harness the power of simulations to identify potential failure points and risks that need to be accounted for.
Unlocking the Potential of Machine Learning in Supply Chain Planning
In an increasingly complex environment with disruptions and growing uncertainty, companies are continuously challenged to quickly analyze large amounts of data. As supply chains grow more interconnected, advances in AI/ML are helping organizations improve the quality and speed of decisions with a higher degree of automation. This means supply chain leaders can focus on strategic initiatives that drive growth while staying ahead of rapidly evolving market conditions.
As with the NFL, more supply chain data is available than any time before, allowing companies to move beyond analyzing basic KPIs such as volume, cost and service level to evolve into contextualizing data across the broader supply chain ecosystem.
Additionally, ML can be used to create self-learning models. This can be especially useful when embedding AI to translate between analytics and how individuals both consume and perceive data. Just like the representation of players’ metrics and projected performance, supply chain leaders can leverage AI/ML to analyze the impacts of moving from single to multi-sourcing, to increase capacity, to add labor or shifts or to hold more safety stock.
The power of data and analytics has outpaced traditional supply chain processes at many companies. Leveraging advanced systems and AI allows teams to accelerate strategies and reap tremendous benefits to the business by:
- Taking a ‘look back’ to identify the indicators of risk, uncertainty and change.
- Evaluating products, customers, vendors, resources and more to highlight the characteristics.
- To create insights and connections between seemingly unrelated data elements.
And then use ML to continually monitor those indicators to transform the outcome of planning and make changes in your supply chain that address the shifting conditions, manage risks and maximize opportunities.
We’ve come a long way from spreadsheets and using basic data elements to derive insights and drive the plan forward. Supply chains can learn a lot from professional sports and the ability to derive greater, more valuable insights. To do so, we need to go beyond the basic thought-process, beyond traditional metrics to look at other factors that could impact operations. Sports analysts may have seen Haason Reddick as an exceptional player, but it was the ability to look at all the various factors that could impact his performance that started to paint the real picture and highlight what we all already know– business, as in sports, is about more than just the individual. It is the team, the factors that surround us that also contribute to our success or stumbles.
For supply chain, it’s past the time to start using data more wisely.