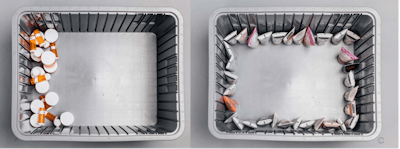
For the first time ever, AI robotics are being widely deployed in warehouses across industries — apparel, e-commerce and consumer goods, to name a few. In these facilities, artificial intelligence (AI) can enable robots to achieve human-level autonomy when it comes to pick-and-place use cases such as order picking, sorter induction, putwall, parcel induction, etc. In fact, industry analysts predict that the number of AI-powered robots in warehouses will grow from around 2,000 today to tens of thousands over the next few years. The ability for modern, deep-learning-based AI to continuously learn and adapt allows robots to autonomously handle the virtually infinite variability that exists within the supply chain.
But, not all AI robotics solutions are created equal. The real-world efficacy of the software “brain” powering the robot can vary, and assessing this new technology is complex:
● How do you estimate real-world performance vs. the generic claimed results shared by vendors?
● How well can the software adapt to changes in object type, environment, use case, etc.?
● How do the above affect the economic and operational viability of adopting such a solution?
To answer these questions, a rigorous performance-based assessment is required that documents the test process, the parameters to measure and how the results should be analyzed. A well-defined evaluation plan will ensure that your test determines the AI robotics software's out-of-box performance, learning speed and potential to adapt over time. As you consider designing your own assessment, take into account the following key considerations.
Measure autonomy
For warehouse applications, autonomy is defined by a robot’s ability to successfully perform tasks without requiring intervention by a human operator over a long period of time. Some key things to observe and measure system autonomy include:
● Throughput. What is the speed at which the robot can autonomously perform tasks (e.g. pick up an object from a bin and place it onto a sorter)? This is usually measured in picks per hour (PPH).
● Accuracy. What percentage of times does the robot successfully perform a task?
● Intervention. How often does a human operator need to intervene because the robot cannot complete a task, such as not being able to pick up an item or dropping it on the ground?
● Recovery. How well is the software able to recover from failure and break deadlock autonomously (e.g. it does not repeat failed grasp actions and uses perturbation instead)?
Test for variability
A virtually infinite number of variations exist when it comes to the type of objects and scene a robot is interacting with during pick-and-place tasks in a warehouse. To assess the resiliency of AI robotics software to handle such variability, your tests should include:
● Challenging objects. Based on inventory, test the robot’s ability to handle objects that vary in transparency, texture, shape, flexibility and size.
● Challenging scene. Present various, unstructured scenes to the robot, such as objects in a bin placed chaotically, packed tightly, placed in divided compartments within the bins, placed along the sides of the bin or on top of each other.
Test learning speed over time
One of the key aspects of what makes neural network-based AI the ideal solution for warehouse pick-and-place applications is its ability to continuously learn and adapt. However, learning speed is often overlooked when assessing AI robotics software. So how long should it take the software to learn?
For modern-day AI software, 72 hours should be sufficient to incorporate new data and provide an improved model. The software provider should wait 72 hours after the initial “out-of-the-box” tests and then perform those same tests again to measure the improvement. Much like an associate, the robot brain should learn from its successes and failures and continue to improve.
Taking the next step
When assessing AI robotics solutions, your primary questions should be, Can this solution perform at a human level in terms of speed and accuracy? Can it adapt and learn from new circumstances? Will it address my current real-world warehouse use cases? Will it address future use cases?
The potential of AI robotics to transform materials handling is just at the beginning of a significant inflection point.