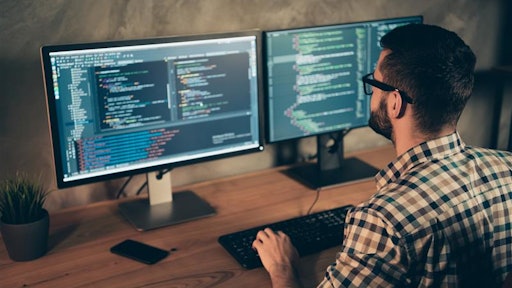
As the amount of available data in the transportation and logistics industry has grown exponentially in recent years, the industry has been challenged with figuring out how to use that information to create value via actionable insights.
With the influx of sensors, telematics data, video systems, back-office platforms and more, supply chain companies generate billions of data points every day. All of this available data is a treasure trove for data scientists, who can apply algorithms to data sets in order to predict – and change – the future through predictive and prescriptive analytics.
Predictive analytics uses data from past events to make a prediction about the future, based on the current state of a situation. Prescriptive analytics takes that prediction a step further by making a judgment about what has worked (or not) in the past, to recommend an action to change a future outcome.
In simple terms, the distinction between the two is that predictive analytics gives you “what will happen,” and prescriptive analytics helps you take action to change what will happen.
As transportation software has become more sophisticated, the number of solutions and features utilizing predictive and prescriptive analytics has grown. These analytics are used for practical applications such as asset maintenance, load matching, fatigue monitoring, safety and risk analysis and more.
Working in tandem, these solutions are paving the way for a more connected supply chain that will enable stakeholders to work together more collaboratively, ultimately creating efficiencies and reducing costs.
Show your work
Data analytics in the logistics space has come a long way in recent history. Less than two decades ago, it was common for data to be fed into what could be called “black box” technologies, with no visibility into why an algorithm arrived at a certain conclusion. At that time, a company simply had to trust that the science behind the models was accurate, and it could be difficult even for a data scientist to explain why the system worked.
Back then, it was much more common to see solutions based solely on predictive models, which then required staff to make a call on next steps based on the algorithm’s prediction.
In the past, predictive and prescriptive modeling was sometimes seen as something mysterious, like reading a crystal ball, but these days, it’s been taken to an operational level where you don’t have to be a mathematician or data scientist in order to understand how a solution arrived at a conclusion. The current name of the game is “show me your work!”
Making better decisions
As predictive and prescriptive analytics have evolved, the focus is much more on developing solutions that pull in a variety of complex data sources and then prescribe recommended actions, and there is a stronger emphasis on sharing “why” a prediction and recommendation was made, so all parties can understand.
This approach helps uncover value across the entire supply chain – sometimes it’s a simple case of “you don’t know what you don’t know.”
For instance, for shippers matching loads to available carriers, it’s no longer sufficient for a solution to analyze a previous match and simply say: “This carrier underperformed with this load.” Now, we have predictive models that can get to a deeper level to say, “This carrier underperformed, and here’s why.” Perhaps they did not have enough assets in the area to account for a weather delay, for example, knowing this information allows companies make more informed business decisions in the future.
From the carrier’s perspective, they can analyze the same situation and make a decision about if bidding on that shipper’s freight in that region is profitable for future bids, or if they would deploy additional assets or perhaps assign the route to a different driver who is more experienced with certain types of weather or areas. A prescriptive model could help determine what that recommended next action would be.
When functioning at their best within the transportation industry, algorithms can provide recommended actions based on past events and current circumstances, but ultimately, the action must be taken by a person. Predictive and prescriptive modeling truly enables humans to play to their strengths and take action, while letting computers take on the more mundane, time-consuming or number-crunching tasks.
The more, the merrier
One of the classic concepts in data science is that a small sample size makes it difficult to gauge the accuracy of a model. So, when it boils down to it, when more companies use analytics tools, more data becomes available, which in turn increases accuracy and expands the capabilities and potential uses for analytics-based solutions.
As more and more shippers, carriers and third parties – really, any stakeholders in the supply chain – participate in the connected supply chain concept, the more innovative, unique and useful solutions vendors can develop, providing more accurate predictions and prescriptions.
At the end of the day, there is really no limit to where we can apply this technology, so the time to begin participating in the connected supply chain of the future is now.