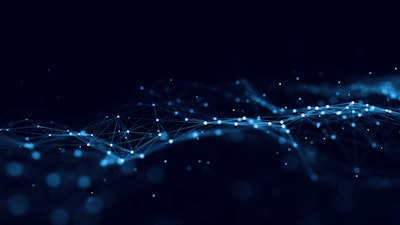
Saying a business runs on ‘governed data’ is like saying a building is built on ‘real concrete’ – it should already be understood. And yet, many manufacturing companies are working with data that is not managed under one platform of defined rules and policies to ensure its accuracy, consistency, security and usability, thus putting overall business integrity at risk.
As the fast-paced manufacturing industry looks to scale into Industry 4.0 and beyond, it’s imperative to establish a strong foundation built on data. Once the initial data auditing process is complete, teams can then focus on unlocking greater operational efficiency through innovations like advanced analytics, digital twin simulations, edge computing, robotics, sustainability systems and even on an even more substantial level, AI.
AI is key for maximizing overall efficiency and achieving more advanced transformation. When implemented correctly, AI will have a mutually beneficial relationship with various technologies, including machine learning, neural networks, natural language processing, and robotics. Here’s how manufacturing leaders can bolster their data governance and infuse AI to elevate current technology systems and business functions.
Break Down Bottlenecks with Predictive Maintenance
For manufacturers early in their AI journey, leveraging AI for predictive maintenance is a great place to start as it delivers almost immediate returns. AI algorithms lie on top of sensor data and software to analyze trends and predict when maintenance is required, reducing downtime and preventing costly breakdowns. Several departments will benefit from this automation as information is seamlessly and swiftly shared by a data platform – outside of the maintenance room.
For example, HR or plant managers can proactively adjust for the influx of reallocated work, and procurement can anticipate raw material needs based on the revised backlog. Additionally, sales personnel will be alerted to any phone line issues and can update their lead times accordingly and production schedulers can proactively look at other equipment or factories.
Production scheduling is a particularly complex task that involves coordinating various resources, such as machines, labor, and materials. AI can optimize production schedules by analyzing governed data and identifying the most efficient allocation of resources to minimize delays and maximize output. Governed data ensures that the information used for scheduling is accurate and up to date, leading to more efficient production processes. Should any of that data fed to AI be incorrect, the resulting decision will fall well short of its desired outcome.
With the addition of AI and automation, departments can achieve true workflow adaptability and efficiency, and governed data ensures that the data fed into AI models is reliable and consistent, leading to more precise predictive analytics.
Automate to Boost Quality Control
Quality control is another area where AI can help, as AI systems can inspect products in real time, identifying defects and ensuring consistent quality without human intervention. It accomplishes this by comparing various aspects of a product or production stages against a master schematic or value. Errors can then be caught before a substantial lot is produced or rejections are seen by the customer or at the job site. Another advantage is knowing exactly where the error occurred, which saves considerable time from having to audit each stage to pinpoint the faulty process.
AI-driven quality control systems rely on large datasets to identify patterns and anomalies. Governed data ensures that the datasets used are accurate and comprehensive. This enhances the AI system’s ability to detect defects in real time, reducing waste and improving product quality. For instance, in a production line, AI can analyze images of products to identify defects that are not visible to the human eye, ensuring that only high-quality products reach the market. If a part’s dimensions are incorrect or outdated from the real-time version of a single truth, AI cannot do its job effectively.
Put the “ai” in Supply Chain
Manufacturing organizations can boost supply chains by leveraging AI to forecast demand or adapt to sudden changes in order logs, optimizing inventory levels and streamlining logistics. It can also help find and onboard new suppliers when it detects your current supply chain is at risk of running low on compliant materials. Interestingly, AI can not only adjust according to internal data from factories, but can also leverage public data, such as geopolitical, health, and shipping data, to anticipate delays or closures on a regional scale. Providing internal optimization—based on external factors—is one of AI’s most valuable capabilities.
By analyzing governed data from various sources, such as suppliers, inventory, and sales, AI can predict demand, manage inventory levels, and improve logistics. Governed data ensures that the information used for these analyses is accurate and up to date, leading to more efficient supply chains overall. For example, AI can forecast demand for raw materials more accurately based on a governed bill of materials (BOM), ensuring that inventory levels are optimized to meet global or regional customer demand and production needs without overstocking.
Analyze Data to Support a Greener Future
AI can play an important role in monitoring and optimizing energy usage, reducing waste, lowering operational costs, and supporting overall ESG goals. The data collected by AI can also aid in reporting for compliance or regulatory audits on carbon footprint and greenhouse gas emissions.
Manufacturing processes can be highly energy-intensive, and conserving energy usage is crucial for reducing operational costs and negative environmental impact. AI can analyze governed data on energy consumption and production processes to identify opportunities for energy savings. For example, AI can adjust machine settings and production schedules to minimize energy usage during peak demand periods, reducing energy costs and improving sustainability. Aligning these to a master asset or location, allows an enterprise to accurately meet established requirements.
Get Set Up for Success
It has become clear that the future of manufacturing will be increasingly data-driven and AI-powered. While the integration of AI and governed data to collaborate with human decision-making (Industry 5.0) offers significant benefits, several challenges must be solved. Here are common data obstacles and how manufacturers can overcome them:
- Data Cleansing, Integration, and Scalability: Integrating data from various sources and ensuring its accuracy and consistency can be difficult, especially when older legacy systems are involved. Effective data governance practices are key to mitigating issues before the second phase of standardization and ensuring that the sharing of the data is seamless between divisions, locations and platforms.
- Data Security and Privacy: Protecting sensitive data from unauthorized access and ensuring compliance with data privacy regulations is critical. Robust data governance frameworks and security measures are necessary to safeguard data.
- Skill Gaps: Implementing AI technologies requires specialized skills and expertise. Organizations must invest in training and development to build the necessary capabilities or partner with leaders in the space that can provide a data hub of governed data to feed various Large Language Models (LLMs).
Over time, the integration of AI with governed data will become more sophisticated, enabling even greater levels of operational efficiency. While challenges will always exist, the benefits of AI and governed data are clear, offering a path to enhanced productivity, reduced costs, and improved product quality. For manufacturers looking to keep pace and compete on a global scale, high-quality data and AI will be key.