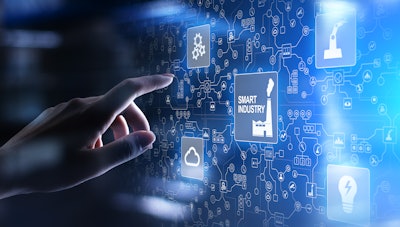
As more businesses move towards complete digital transformation, the amount of data generated increases exponentially. As just one measure, IDC predicts there will be 175 zettabytes of data worldwide by 2025. Properly analyzed, such volumes of diverse structured and unstructured enterprise data can reap tremendous insights for enhanced business decision-making across industrial production and supply chain environments.
Hence the rise of decision intelligence, which Gartner defines as a category of technology-enabled decision-making techniques that bring together multiple traditional and advanced disciplines to monitor, model, optimize, execute and maintain decision models and processes. The challenge is that decision intelligence relies on managing data volumes so large as to be impossible for humans to manually process and analyze.
Let’s explore how enterprises can optimize their existing digital operations with AI and automation that can help mature capabilities of digital intelligence, including what’s required, what the process looks like, and the tremendous benefits derived from manufacturing operations, supply chain logistics, and more.
Decision intelligence requires navigating data at scale
Organizations that embrace digital transformation know, first and foremost, that they’re dealing with a challenge of scale. Navigating tremendous new volumes and varieties of data is the key to survival and growth, and success is measured by the ability to leverage this data for the practical business context and actionable decision support. This is especially true for key areas such as supply chain, procurement, organizational resource allocation, and task management.
Decision intelligence is made possible when industrial production and supply chain managers leverage technology aggressively to better observe and analyze data; tease out causes and effects; discern how actions lead to outcomes, and then share business intelligence and recommendations for decision support. Advanced analytics allow teams to closely evaluate options and weigh the risk-reward dynamic for each potential decision.
It’s a compelling value proposition, which is why Gartner named decision intelligence one of its top technology trends for 2022 and predicted that more than a third of large organizations will be using advanced decision intelligence technology by 2023. However, this projected growth relies on the success of underlying modernization efforts of data architectures for enterprises to handle such vast amounts of data that feed the complex modeling processes required for decision intelligence; and this can be easier said than done.
That’s because, behind every actionable, evidence-based recommendation is a vast process of gathering and analyzing data around new product development, cycle times, change management, product quality, supplier management, manufacturability, regulatory compliance, and more. Given the speed and scale of the enterprise production and supply chain environments in which this analysis is happening, decision intelligence relies on greater adoption of artificial intelligence (AI) and automation in order to succeed.
The importance of AI, automation, and digital twin modeling for decision intelligence
AI and autonomous systems capabilities are changing the industrial automation landscape. Expanded adoptions and brisk implementations by leading manufacturers are driving global industrial AI toward becoming a $102 billion market by 2026. But how can these advancements in technological capabilities be optimally leveraged specifically to support decision intelligence?
A big part of the answer lies in what’s known as digital twin capabilities. A digital twin is a digital representation of a physical process or machine that replicates its behavior as it undergoes changes in its environment. Traditionally, digital twins have been developed using explicit programming based on knowledge of the “first principles” of the system. These first principles models utilize physical and process equations to simulate and analyze the supply chain interactions within the system. Building and calibrating the first principle models can be a long and expensive process. Decision support systems built based on the first principle models are useful to a certain degree, but they are inadequate due to the lack of capabilities to learn from past experiences leveraging the wealth of historical data. With the advancements in AI/ML technologies, we now have the capabilities to augment our digital twins with machine learning, enabling them to continuously learn and even more important to adapt to new situations as changes manifest. This is important because these digital twins can utilize past data to derive nuances out of data that extend the knowledge that engineers already have. It also means that it’s not just enough to understand the first principles of a complex process. You also need to be able to rely on historical observations that can be used to derive an even more robust understanding of what is happening beyond what a team of engineers can do independently.
Digital twin models capture business context and operating conditions through a variety of sensors, meters, and other devices to measure system performance, the condition of assets, and any anomalies in operations. In real-time, this data feeds into control algorithms that proactively identify where adjustments are needed and where problems may arise. The most advanced digital twin models can leverage closed-loop AI Operations (AIOps) capabilities to perform auto-resolution on many issues.
Decision support is further streamlined by accessible visualizations for users to monitor changing conditions and determine when and where human intervention may be needed. All the while, the ongoing flow of real-time data from assets and systems charts impacts on system or asset performance for a continuous improvement loop that helps optimize operations on an ongoing basis. Furthermore, digital twin modeling can be further advanced to a point where robust virtual models can be developed for each production and supply chain asset within a system. This typically means significant reductions in model building costs to optimize throughput by utilizing a digital twin that continuously learns and adapts for effective decision-making.
In an autonomous factory, this repeatable methodology can be used to model and optimize key performance characteristics anywhere in the production or supply chain ecosystem. Furthermore, the same methodology can be used to connect various individual autonomous systems and align them for a “system of systems” level of decision intelligence across the whole manufacturing and supply chain operation.
Conclusion
Organizations that succeed in implementing decision intelligence enjoy many benefits that range from greater efficiency and reduced downtime in operations to enhanced product quality and streamlined compliance and reporting. As AI and autonomous systems scale across the spectrum of factory and supply chain operations, the data-driven insights derived from a consistent decision intelligence methodology can deliver increased throughput, radical quality improvements, and cost reductions across a manufacturer’s entire service value chain.