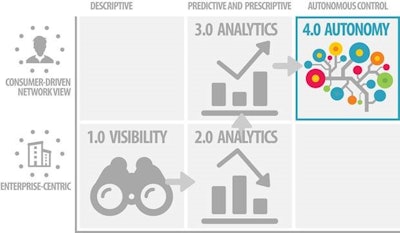
Global supply chains have become even more complex, involving a lot of businesses that must interact to bring us the goods we use and consume.
In the past, businesses had created Supply Chain Control Towers to patch together the different functions and disparate parties in their supply chains. Capgemini Consulting defines a control tower as “a central hub with the required technology, organization and processes to capture and use supply chain data to provide enhanced visibility for short and long-term decision making that is aligned with strategic objectives.” However, new architectures for control towers are going one step further by leveraging innovations in artificial intelligence (AI) technologies to achieve unprecedented levels of performance.
The Evolution of Supply Chain Control Towers
The earliest control towers served as consoles, which provided visibility for managing the supply chain for a given business. In recent years, these early “1.0 Control Towers“ have evolved to include predictive alerts and prescriptive decision-support capability.
Control Tower 2.0 systems took an “inside-out” view of the world. This view placed one’s own business at the center of the control tower, looking out to immediate suppliers and immediate customers, while everyone else was treated as an afterthought. This created several limitations in terms of reduced operational reach, an explosion in point-to-point integrations, decision-support, and the inability to coordinate planning and execution across trading partners.
Control Tower 3.0 took an outside-in perspective, which placed the business and its trading partners as part of a multi-enterprise network, working together to serve the consumer. These true consumer-centric networks provide a digital network platform that brings people, process and technology from multiple independent businesses. More importantly, it allows all to execute together in lock-step around the consumer’s needs and deliver superior performance.
According to Nucleus Research, customers who adopted a customer-centric network realized a 56 percent increase in inventory turns and a 38 percent decrease in safety stock holdings on average. The research also found that customers benefit from greater visibility, better coordination, and superior optimization within their extended supply chain across multiple tiers of trading partners and suppliers.
Control Tower 4.0
Now, AI technology is disrupting the status quo once again by enabling a performance leap that is virtually impossible to achieve with traditional software. These AI-enabled Control Tower 4.0 solutions move beyond just decision-support to include decision-making and autonomous control. The network is predictive, resilient and capable of running itself.
For example, at one retailer, intelligent agents are monitoring consumer purchases at stores to continuously anticipate, sense and respond to real-time changes in buying behavior. Agents automatically adjust replenishments based on supply demand balance, and the company has already seen a 40 percent improvement in forecast accuracy.
Another example involves an e-commerce retailer who was struggling with the supply chain due to its rapid growth. In one quarter alone, the organization saw a five-fold increase in number of products, six-fold increase in number of customers and an eight-fold increase in number of suppliers. Despite this, they were able to maintain 99.5 percent on-time delivery performance without adding headcount to their supply chain team by on-boarding to an intelligent consumer-driven network. By deploying a touch-less fulfillment process, their staff achieved these results by focusing on only the most critical issues and engaging proactively with impacted customers.
A New Functional Architecture
Today, the 3.0 networks act as a System of Engagement (SOE) that orchestrates execution over the many Systems of Record (SOR), people and things to provide joint transaction and execution services in real-time. Unlike traditional enterprise-centric systems, the network becomes a digital platform that AI technologies can leverage to provide scalable and efficient decision-making.
New layers of agent-based systems, called Systems of Intelligence (SOI), have emerged that sit on top of the SOE and add even greater functionality. These systems use trained decision models to make rapid optimized decisions during execution with up-to-the minute information across the network and on-the-ground local context about people and things.
Above the SOI is yet another layer called the Systems of Cognition (SOC), whose function is to learn and adapt to the decision models in use by SOI, so the network can get smarter over time. Each of these technology elements serve a valuable function.
System of Engagement
SOEs require a multi-party network as it bridges the physical world with the virtual. Digital twins represent every actor, such as people and things, while physical events and multi-party transactions trigger real-time changes in state in the digital world. Digital models, known as state machines, control the possible activity and process lifecycles.
Multi-party master data management services provide a shared vocabulary across the network. Business social apps and conversational user interfaces provide means for both structured and unstructured data to be gleaned from the physical world, put in context, interpreted and acted upon.
Finally, blockchains, or other permissioned ledger technologies, maintain a secure audit trail of the business transactions across all parties. The SOE provides real-time governance and orchestration of multi-party workflows across the network.
Systems of Intelligence
At the heart of the SOI layer are intelligent agents and a transaction grid. Agents don’t just plan; they execute, and they act independently within a sub-network to perform micro-tasks such as “micro-optimizations” with specialized purpose and goals.
Agents have predictive intelligence and they act very fast and communicate with each other as needed, working directly on transactions. At any given point in time there could be millions of agents “in flight.” It is the job of the transactional grid to manage this.
Supervisory agents operating on the transactional grid orchestrate agent activities. They can rollback and retry agent reactions. The grid can scale horizontally and grow as more companies, items, facilities, and/or orders are added. The cloud network only has to add more servers to scale. This is in stark contrast to traditional planning engines and in-memory computing technologies, which use vertical scaling and max out quickly.
The agent-based grid enables smart decisions, fast, in a highly scalable architecture.
System of Cognition
Finally, a third layer called SOC has emerged, which consists of agents that can learn from experience and modify the behavior of the SOI agents.
These systems use a variety of AI technologies, such as deep neural networks, to perceive patterns, diagnose root causes of anomalies and learn from Big Data to train digital memories.
Learning agents essentially observe the decisions and results from the SOI agents and modify their underlying assumptions and models. Machine learning algorithms improve with data, which is what the SOE network readily provides. The more the network runs, the smarter it gets.
Getting Value from AI
AI is starting to make its presence felt in supply chains, as the benefits realized by early adopters are significant and include the following:
1. Automation—The lowest hanging fruit for AI applications has always been to automate the most routine and mechanical tasks that humans do, so they can be free to focus on higher value tasks. The answer is not necessarily to replace people with robots, but rather to automate some of the tasks in order to get the most effective outcome.
2. Expertise for All—The second and natural by-product of building decision models is that they can identify insights that can help more people to replicate the insights and trade-offs that are uncovered. Known as “institutionalizing knowledge,” this is like having an expert looking over your shoulder and guiding you. The expert may not be as close to the specific problem, and it may not have all the subtle meanings, but it can offer you guidance based on what worked well and what to watch out for from years of experience (or in this case: a lot of training data).
3. Improving Decision-Making—The third way AI creates value is by helping everyone – experts included – make better decisions. When asked how he has leveraged new Control Tower 4.0 to bolster the supply chain, Alan Pendleton of Republic Wireless recently said it best: “We want our supply chain team to spend more time thinking and a lot less time doing. We want them to be strategic thinkers, decision makers, negotiators, and ultimately, advocates for the customer experience – not order expediters.”
So, in the end, AI is not myopically about removing people, rather it as a way for organizations to extend their reach, improve lives and help make smarter decisions, faster.