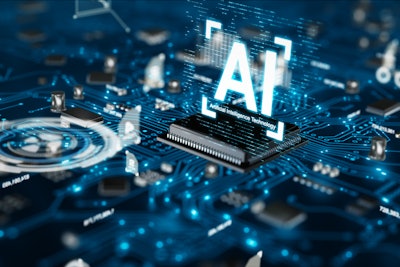
A gap is growing between business and IT leaders. Recent reports suggest that business leaders are losing faith in IT. This is attributed to a lack of communication and collaboration between the two factions. While IT and business leaders are both focused on helping the company succeed, it is proving difficult to remain aligned in the rapidly changing environment. IT leaders have been inundated with questions regarding advances in AI tools. The promise and perils of AI advancements has most industries on edge, and the fear of missing out is extremely high. Trying to keep up with the development and evolution in this area is taking a disproportionate amount of the IT leadership team’s bandwidth. This environment is definitely contributing to the communication challenges, but other issues need to be addressed as well.
One key issue driving the gap between IT and business leaders is a lack of a clearly documented strategy for leveraging AI to resolve business challenges. Glitzy AI demonstrations are designed to be thought-provoking and to stoke the possibilities of disruptive advancements. When entering the AI marketplace to fix one issue, it is easy to find yourself pursuing a future state that completely eliminates issues with the existing workflows. Those entering the AI marketplace must segregate what “could be” from what “can be.” Every industry needs to work both disruptive future state and optimized current state opportunities in parallel. In too many instances, the novel opportunities that AI enables are prioritized at the expense of refining existing processes and workflows. This often leads to Business leaders being underwhelmed by AI investments as the requested improvements to existing workflows with obvious benefits are deferred.
Competing objectives need to be discussed and balanced. The development of potential future states cannot completely stall improvements in current workflows. Near-term improvements for existing workflows need to focus on time-consuming tasks where AI capabilities can easily streamline the process. These near-term improvements have the advantage of delivering immediate performance improvements. The impact of the changes is easily measured using existing KPIs. These savings can fund more speculative or ambitious investigations.
That said, disruptive new workflows do deserve immediate attention. Like any new structure, these new workflows require a solid foundation, often the most time-consuming task. Supply chain optimization is a prime example of the challenges of unlocking AI’s potential. The opportunities to streamline analysis and execute transactions are very fertile ground for AI. It is easy to skip a few chapters ahead and envision AI agents effectively managing these workflows. Future state workflows where a few senior supply chain leaders effectively manage the limited exceptions detected by a team of AI agents are enticing. As I’ve discussed in prior posts, realizing this vision involves several incremental steps that can’t be skipped. These foundational elements include data fidelity, robust categorization, algorithm-based decision trees, and exception detection/escalation (i.e. allocation decisions that are not purely mathematical and require human input).
The old adage of “garbage in, garbage out” couldn’t be more true when discussing AI solutions. AI supply chain agents are going to rely heavily on ERP data. The amount of metadata associated with each item (e.g. price, demand, quantity, and order policy) is both impressive and daunting at the same time. There are roughly 20 data elements at a minimum, but the average is closer to 30. The number of SKUs managed by a manufacturing facility varies widely, but they routinely range from thousands to tens of thousands. In these environments, the challenge of maintaining accurate data is readily apparent. Even maintaining 99% accuracy for a facility with 10,000 SKUs with 20 data elements would yield 2,000 erroneous data elements. These errors then compound since decisions made for one item often impact decisions on other items used to complete the end item assembly. In many industries, Six Sigma levels (99.9997%) of data accuracy will be required to ensure the conclusions reached by AI agents can be trusted and potentially fully automated.
Given the challenges to achieving these levels of data integrity, one of the first applications of AI will be to cleanse and verify the data it will use to make decisions in the future. Remember that achieving very high levels of data accuracy is just the first step in your journey. You’re still a long way from unleashing autonomous AI agents. You still have the sizable tasks of developing categorization rules and robust algorithms involving numerous variables. Each alternative has pros and cons that impact a wide range of internal and external customers. Coalescing the tribal knowledge of your organization into agreed algorithms is one of the most challenging (and ultimately rewarding) endeavors.
The final steps typically involve integrating these ‘synthetic agents’ into the “real world.” AI agents will need to interface with humans for the foreseeable future. Requests to amend and update thousands of delivery lines on hundreds of purchase orders will be met with resistance from human counterparts. Nuanced decisions involving allocating limited supply to competing customers is another area where logic only gets you so far. Recognizing when decisions require human interaction is one of the last steps in the process and will require a lot of trial and error.
In this supply chain scenario, many Business leaders are making relatively general requests to their IT counterparts like the “opportunities to streamline the procurement process”. Recurring tasks like issuing requests for quotes and generating purchase orders are areas where generative AI can easily take some of the load off the buyers. Narrowly scoped requests like this can lead to the problem of those researching solutions over-indexing to more ambitious future states like AI agents. To help prevent these miscommunications, requests should be coordinated and include both near-term and long-term goals to reflect the overarching strategy. In this case, in addition to using Gen AI solutions to streamline the existing procurement process, steps to automate data health checks and master data correction would be worked in parallel.
The disconnect between business and IT leaders regarding how to leverage AI is not really surprising. The environment is (and continues to be) very fluid. Frequent communication is critical to ensure that business leaders get the tools they expect and that IT leaders do not waste valuable resources investigating solutions that do not address the critical next steps on the roadmap to their vision of the future.