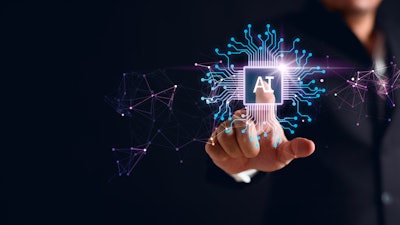
Containers and reusable packaging like pallets or plastic crates are in integral part of many supply chains and industries, including food and automotive. Imagine you are in a depot, trying to get an overview of your containers and reusable packaging assets. You pull out your smartphone and ask your voice assistant: "How many IBC containers are available in this depot?" The answer appears immediately on the display and is read out by the voice assistant. Precise demand forecasts for this container type and optimized suggestions for transport orders follow shortly afterwards. This scenario, which was still science fiction a short time ago, is now reality thanks to advances in artificial intelligence.
Many companies have already had positive experiences with AI in marketing or dynamic pricing, for example. Gradually, however, companies are also beginning to focus on optimizing their supply chain processes. We will therefore look at a concrete example: container and reusable packaging management, a logistical challenge that ensures that each site has the right amount of assets at the right time by planning for future demand and adapting to real-time supply chain challenges.
Juggling with Blindfolds On
Many manufacturers, retailers and logistics companies operate asset pools, some of them containing a large number of very high-quality returnable assets. Tens of thousands or even millions of crates, ULDs, containers and other reusable assets move through a variety of locations such as suppliers, manufacturers, logistics companies, distribution centers, retailers, cleaning facilities and - if things go well - circle back to the suppliers.
If things go well!? Today's logistics are challenged by volatile markets and densely interconnected supply chains: although more and more data are being collected throughout the chain, companies still rarely bundle this data into an effective decision-making system. The actual and virtual stock of returnable assets often do not match. Moreover, different software systems are used to manage the incoming and outgoing assets without communicating with each other. Thus, incorrectly allocated containers will hardly be noticed.
With that lack of transparency, spontaneous deviations, day-to-day delays and time-sensitive assignments can jeopardize the entire operations. If, for example, a customer with a ‘play-it-safe’ mentality orders a surprisingly large number of empty containers these will be tied up in safety stock but may be missing at the production site of another company needed for a time critical move.
AI to Provide Clarity
Even if all returnable assets are regularly recorded and visualized in a central platform using modern Auto-ID and telematics solutions, the bottlenecks described above are by no means always apparent given the vast amount of container and transaction data. How can dispatchers possibly have a complete overview of the status, locations and routes of hundreds of thousands of containers in a supply chain cycle? What condition are these assets in and where exactly are they needed and at what time and quantity? Has the planning included all orders as well as damaged containers, cleaning and redistribution times?
Optimized, demand-driven distribution is therefore not just a question of transparency, but also of the ability to make sound decisions quickly. This is where artificial intelligence comes into play. Algorithms are able to calculate optimized recommendations for action in real time or even decide and act on their own if required, for example to generate transport orders.
In order to precisely forecast demand for containers and to identify possible inconsistencies (e.g., over- or under-stocking, violation of geographical fences or faulty Auto-ID hardware), AI can consider a wide range of information: manual input from dispatchers (so called ‘domain knowledge’), data from external systems (e.g. ERP and PPS systems), predefined rules such as minimum and maximum stock levels, range analyses and historical data. All relevant factors can thus be taken into account when making forecasts and decisions.
Continuous Improvement Through Machine Learning
One of the most powerful functionalities of AI is the ability to recognize correlations and patterns from a vast amount of data and sources and to derive useful knowledge and recommendations for action from the insights gained. By integrating machine learning technologies, it is possible to learn iteratively from the data. These technologies take a bird's eye view and identify previously unrecognized patterns within processes without being told what they are looking for.
An example: A customer repeatedly accumulates high safety stocks well in advance. In this situation, a human dispatcher would often delay the empty container order by a few days in order to achieve good overall results despite the change in planning. Over the years, a dispatcher has learned the stock limit at which reordering becomes necessary. A machine-learning system could observe this behavior, link it to other findings (e.g., "When is the customer likely to reorder empty containers?") and, after a training phase, automatically adjust the orders.
Hybrid-AI Pushes for the Next Level
For all this to work, it’s important to not rely exclusively on such data-driven AI methods, as prominent as they may be nowadays. Instead, knowledge-based methods of AI such as search and optimization algorithms from operations research or fuzzy logic should play their part in a hybrid approach (Hybrid AI). With this it is possible to map expert knowledge regarding business processes, behavioral patterns, planning goals or constraints through mathematical decision modeling. Algorithms can then quickly browse through all potential decision scenarios. Considering countless variants of how to act in any given decision situation, the AI is able to decide the best course of action for the task at hand, and then execute or suggest it to the user.
This technology makes it possible to manage complex container and reusable packaging networks with a high level of planning and service quality. It can not only send a warning to users in the event of bottlenecks, but also ensure that the required quantities are available in the right place at the right time by automatically balancing containers in real time while also keeping important metrics like transport cost in mind.
These examples show the enormous complexity that AI can help to overcome. Humans, of course, will stay in the loop. As with previous waves of automation, people will be relieved of repetitive tasks and can concentrate on exception-based handling and disruptions.