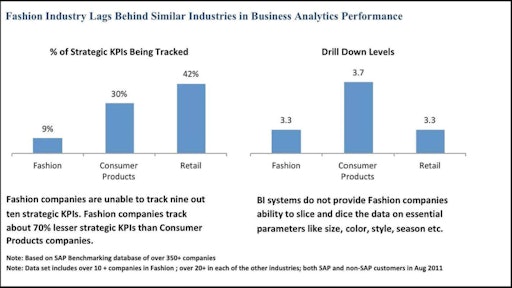
Picture a clothing store where the apparel is arranged according to its popularity on social media. Or a design studio which creates new clothes for the next season based on real-time feedback received from Facebook and Pinterest. Better yet, imagine a design studio where customers can recommend shades, patterns and colors for apparel and even play with virtual fashion games.
No, we’re not talking about the future. This is happening now. Shopper engagement has overtaken the retail, footwear and apparel (RFA) industry, bringing fashion crowd-sourcing to new heights. And while most businesses in the RFA space optimize data analytics for proactive business growth and ROI, even more will have to open themselves up to technology adoption and new analytic utilization to prepare for upcoming trends while responding to real-time activity in this space.
Getting social with fashion
With the obvious gains that social media brings to retail—from sales promotion to sneak peeks at upcoming inventory—it’s evident that the fashion industry must continue to find new ways to evolve with and engage customers. Manufacturers can no longer impose styles on consumers.
Fashion organizations launch new collections every season in hopes that their styles appeal to consumers to bring in the dollars. But with new aspirations created every moment through the plethora of digital mediums available to the customer, it becomes a huge challenge for design studios to match market expectations. Retailers and fashion giants continue to use Facebook, Pinterest and Twitter to gain ideas and suggestions from customers on everything from designs to retail preferences.
In fact, according to a recent survey from Econsultancy, social media activities are the fourth most engaged activity online as 10 percent of time spent online is spent on social networks. Facebook lists more than 500 million users—and continues to add more than 500,000 new users each day. YouTube records show that more than two billion videos are accessed in a day. And Twitter receives 190 million visitors a month. Such huge numbers gave the fashion industry an enormous opportunity to engage customers on a global scale. And all of this data is then routed to advanced product lifecycle management (PLM) systems that analyze it and help plan merchandising, advertising and seasonal planning.
And in this highly competitive environment—with consumer preferences changing often—real-time market intelligence is a must-have tool. How can a designer predict if paisley is going to work, or what colors are trending? Analyzing conversations on social networks and using that information as business intelligence will help with everything from predicting the preferred mix of colors and sizes, to pricing strategies that work. Retailers such as Marks & Spencer, J. C. Penney, Kohl’s and Kroger increasingly adopted analytics to address operation inefficiencies and cost savings—and with significant results.
Yet, despite these obvious challenges, the fashion industry is much behind other industries in using analytics as a business tool. According to a benchmarking study from SAP, “fashion companies track about 70 percent lesser strategic KPI’s than consumer products companies,” (see SAP Benchmarking graphic).
A new style of analytics
The fashion industry cannot ignore the wealth of relevant information buried inside each social media channel. But in specific technology adaptation, what analytic capabilities may deliver for other retail segments may not bode the same intelligence benefits for the fashion industry. In the case of the grocery industry, technology helped in this space more where past customer preferences and consumption patterns could help predict future assortments and even suggest replenishment levels. This would not work for the fashion industry which does not readily welcome repetition.
New style design decisions are creative decisions but often gut-based with little means to rely on real-time facts making season planning unpredictable. Fishing out cues from past season successes to decide next season designs is a time-consuming process, with few chances of success. In addition, which attribute—fabric, texture or color in swatches—contributes to a winning style is also difficult to gather as sample swatches lie in files not easily retrievable. And with no existing ERP transactions effectively capturing this information, line reviews were usually not backed with facts, making the entire line planning process rather unpredictable.
A combination of style performance analytics and social media analytics is helping the industry foresee dynamic changes and consumer impulses. Several retailers and fashion designers have mined and analyzed social intelligence for real-time feedback on fashion trends to gauge consumer response to their launched or upcoming designs. They monitor social conversations to test reactions to new fashions and even build activities such as apps and games to engage consumers on social media.
The fashion industry is going through a technology revolution with players becoming more open to effective data analysis for business direction; and more data-driven and flexible to take into account real-time opportunities.
PLM tools helped usher a new wave of monitoring product development lifecycles and made the entire process transparent while shaving off cost and time. The library feature stores the entire design elements of the brand which can then be used to design a new style. The level of detail goes much further and helps view a style as more than just an article code and further into design elements.
A Style Performance Analytics solution combines the attribute level information of PLM with data from ERP and uses business intelligence tools to discover winning styles and attributes. Adding fashion elements into a new design need not be gut-based but can now rely on facts to validate if similar inspirations were successful with certain customer segments or geographies. Insights can now predict 'what will sell' and 'what will not sell' and will give contextual feedback on what customers like. Cues can thus be taken to reconfigure designs and the line plan, tweaking assortments to incorporate more styles which fly-off the shelves rather than warm the shelves.
Industry benefits gained
In the move from uncertain, gut-based decisions to fact-based predictable decisions, the industry can thrive from improved revenue; and lower working capital and inventory levels. The customer is now an important part of the manufacturing and product line planning process by testing styles and providing valuable insights into preferences and patterns-which then can translate into products they would actually purchase to benefit the fashion industry.
There’s no doubt that the RFA industry will become more complex and keep evolving at a rapid pace. And with more educated and demanding customers and new customer engagement channels, the industry will need to adapt quickly to satisfy customer needs. Customers demand personalization and involvement in the design process. This is possible only when the fashion industry actually listens to what customers are talking about—and then includes them in the product life cycle.