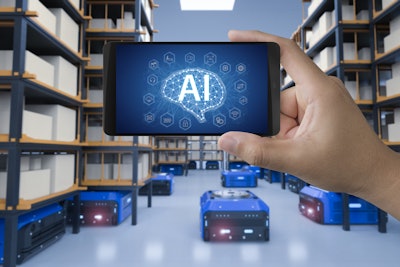
The warehousing industry, which was once run on meticulous manual processes and static spreadsheets, underwent a transformation fueled by Machine learning (ML). While ML has become an indispensable tool, enabling warehouses to optimize operations, streamline logistics, and gain a crucial competitive edge, a new wave of AI technology is now washing the sector: generative AI. A crucial question emerges: how can warehouses effectively integrate generative AI (GenAI) while mitigating its potential pitfalls, such as AI hallucinations?
GenAI, with its ability to generate human-quality text, translate languages and even create realistic images, holds immense promise for the warehousing industry. Large Language Models (LLMs), the workhorses of GenAI, can revolutionize data analysis by uncovering hidden patterns and generating insightful narratives from complex datasets. However, the Achilles' heel of GenAI lies in its susceptibility to "hallucinations" – the creation of information not grounded in reality. These fabricated insights can erode trust in AI systems and lead to poor decision-making. Therefore, for warehouses to successfully navigate the GenAI landscape, a strategic partnership with classic machine learning is paramount.
Classic Machine Learning vs. GenAI in Warehousing
While GenAI holds promise, classic machine learning models backed by years of mathematical development currently hold the upper hand in terms of forecasting accuracy within warehousing. Time-series models, for instance, are far more established than any generative tool. These models excel at analyzing historical data to predict future trends, a critical capability for managing inventory levels, scheduling deliveries and anticipating demand fluctuations. However, the implementation and maintenance of classic ML models can be a complex undertaking, requiring significant technical expertise and parameter tuning. This is where GenAI steps in as a game-changer.
GenAI acts as an intelligent assistant, simplifying the complexities inherent in classic machine learning models. By leveraging its capabilities, users can iterate and refine these models more efficiently, leading to improved decision-making. This empowers decision-makers to act on insights quickly and efficiently, optimizing warehouse operations for maximum impact.
Furthermore, GenAI can act as an invaluable assistant in the development and refinement of classic ML models. The iterative nature of AI allows models to learn and improve with each new data point they process. GenAI can analyze the performance of your ML models, identify areas for improvement and even suggest adjustments to optimize their accuracy. This collaborative approach streamlines the process of model development, allowing warehouses to extract maximum value from their data investments.
Starting Small for Success
To minimize the risk of hallucinations, begin with targeted, manageable AI projects. This allows for close monitoring of AI performance and its impact on specific areas before a broader rollout. Start by optimizing a single process, such as automating picking systems in a designated zone. As you gain confidence in the system's accuracy and effectiveness, you can gradually expand its reach to encompass more complex tasks. This phased approach allows for the identification and mitigation of potential data issues that could lead to hallucinations before they have a significant impact on operations.
Moreover, achieving success with GenAI necessitates a commitment to data quality. The adage "garbage in, garbage out" holds true in the realm of AI. Inaccurate or incomplete data will inevitably lead to inaccurate and potentially misleading outputs from GenAI systems. Therefore, fostering a culture of data quality within the warehouse is essential. This can be achieved through the implementation of robust data collection practices, regular data validation checks and employee training programs that emphasize the importance of data accuracy.
Collaboration is Key
The development and deployment of AI solutions within a warehouse environment should be a collaborative effort. Data scientists, AI developers and warehousing experts must work in tandem to ensure that the AI tools are not only technically sound but also tailored to the specific needs and challenges of the specific warehouse. Regular feedback loops are also crucial. Warehouse personnel who interact with the AI system on a daily basis can provide valuable insights into its performance and identify areas for improvement. By incorporating this user feedback into the development process, warehouses can continuously refine their AI systems and minimize the risk of hallucinations.
The future of GenAI in warehousing is bright. Advancements point to the transformative potential of this technology in supply chain management and business planning. By laying the groundwork now for secure GenAI integration, warehouses can position themselves to capitalize on these future advancements and gain a significant competitive edge, as advancements in other areas - such as robotics - continue surging.
However, for GenAI to reach its full potential, it must be viewed as a partner to, not a replacement for, classic machine learning. By leveraging the strengths of both approaches, prioritizing data quality, and fostering a culture of continuous improvement, warehouses can unlock the true power of AI and achieve a new level of operational efficiency and insight.