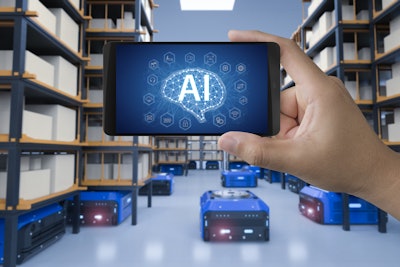
In the ever-evolving landscape of technology, Artificial Intelligence (AI) has emerged as a transformative force, reshaping industries and revolutionizing processes. But it’s still somewhat poorly defined or misunderstood, especially when it comes to the term “generative AI.” In this blog post, we will delve into the distinctions between Applied AI and Generative AI, shedding light on their unique capabilities. We’ll also breakdown how Applied AI, with its real-world applications, can significantly elevate the efficiency of warehouse picking processes.
Defining Applied AI vs Generative AI
Before delving into the practical applications, it's essential to understand the fundamental differences between Applied AI and Generative AI. Applied AI refers to systems that are designed to perform specific tasks, mimicking human intelligence in a narrow domain. These systems are trained on vast datasets and follow pre-defined algorithms to achieve a particular goal. It’s a broad concept encompassing the development of machines or systems that can perform tasks that typically require human intelligence.
On the other hand, Generative AI refers to a type of artificial intelligence that is designed to generate new, original content rather than simply imitating or replicating existing data. This category of AI focuses on creating outputs, such as images, text, audio, or even video, that were not explicitly present in the training data. Generative AI systems often leverage advanced machine learning techniques, such as neural networks, to accomplish this task.
The distinction between these terms lies in the specific functionalities and goals associated with each. Generative AI is a subset of AI, emphasizing the creation of novel outputs, while AI as a whole includes both narrow and broad applications of artificial intelligence technologies.
In the context of warehouse management, Applied AI plays a crucial role in enhancing efficiency, accuracy, and productivity focusing on solving specific problems and streamlining tasks, whereas Generative AI might be more suited for creative tasks like designing new packaging or optimizing warehouse layouts.
Let’s take a look some applied areas in the warehouse where AI-powered solutions can have a real impact.
Using Applied AI in warehouse operations:
- Machine learning algorithms for on-floor travel optimization - Warehouse picking involves the movement of goods from storage locations to shipping areas. Applied AI employs machine learning algorithms to optimize routing, taking into account factors such as order priority, proximity of items, and traffic within the warehouse. This results in streamlined picking routes, minimizing travel time and increasing overall productivity.
- AI–driven slotting results - Dynamic slotting utilizing AI-drive software can lower implementation costs, as it does not require a detailed CAD drawing of the warehouse, as is the case with traditional slotting software. Instead, with AI-based slotting solutions the spatial characteristics and travel time predictions can be automatically learned based on machine learning and activity-level data generated by modern work execution systems. Although there will be some variability, in most cases AI based slotting can deliver 10-20% labor cost savings and increase productivity by 5-20%.
- Workflow orchestration - AI can be used to implement a solution that uses pick rates by zone, labor in zone, totes, and pieces traveling/waiting/being picked in each zone to provide visibility and directives on which totes (order/batch) need to be inducted at which induct point and how much labor is needed in each zone at that time. In other words, optimizing the movement of people and totes through the pick module for maximum efficiency.
- Robotic process automation (RPA) for repetitive tasks - Robotic Process Automation (RPA) in a warehouse environment involves using software robots or bots to automate repetitive tasks and streamline processes, ultimately improving efficiency, accuracy, and productivity. In warehouses, RPA can be applied to various tasks such as inventory management, order processing, picking and packing, and even logistics coordination. By automating these mundane tasks, human workers can focus on more complex and value-added activities, leading to increased efficiency. Software robots can be programmed to automatically update inventory records in real-time. For instance, when new stock arrives, the robots can scan barcodes or RFID tags to identify items, update inventory databases, and assign locations within the warehouse. RPA bots can also monitor inventory levels and automatically generate purchase orders or replenishment requests when stock falls below predefined thresholds. This ensures that the warehouse always maintains optimal stock levels to fulfill orders efficiently.
- Computer vision for object recognition - Computer vision systems, powered by AI, can accurately identify and recognize objects in a warehouse setting. This is especially crucial in environments with a diverse range of products. By swiftly analyzing images or video feeds, Applied AI can enhance the precision of item recognition, reducing errors in the picking process. Amazon's automated picking system utilizes computer vision to identify and locate items on shelves, improving the speed and accuracy of their fulfillment centers.
In the dynamic world of warehouse management, Applied AI stands as a powerful ally, offering concrete solutions to enhance efficiency, accuracy, and productivity. Through the application of computer vision, predictive analytics, machine learning algorithms, and robotic process automation, Applied AI transforms warehouse picking processes, setting the stage for a more streamlined and intelligent future.
While Generative AI has its own creative applications, the focus on solving specific problems in the warehouse domain requires the precision and functionality provided by Applied AI. As technology continues to advance, the synergy between human workers and Applied AI promises to redefine the standards of excellence in warehouse operations.