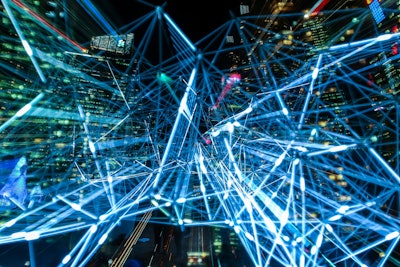
It’s no big secret that leveraging big data analytics has the power to transform a company’s supply chain. Many organizations have now embraced the power of data to make better decisions faster, and the majority have initiatives in place to harness even more data, says John Fullmer, vice president of product management at JDA Software.
In fact, according to a report by Acumen Research and Consulting, the global supply chain analytics market size is expected to be worth $10.7 billion by 2026, growing with 15.5 percent CAGR during the forecast time period. But the supply chain sector still has a long way to go to get the most out of their data.
“The focus is mostly still on internal enterprise data,” says Fullmer, further explaining that many companies are working to archive data about historical performance and plans, but they are still unsure what they are going to do with it. “They’re building out data legs, but it’s still spotty in terms of formalized use of external market data in the supply chain,” he adds.
Where We’re Headed
In terms of data analysis, a fundamental shift took place in 2018 and will continue into this year and beyond. In 2016 and 2017, Fullmer says a lot of projects were focused on delivering insight to a person who was then making a decision about what to do with that analysis. “While the project showed a lot of promise, scaling and operationalizing it to the entire supply chain on a day-to-day basis was a challenge,” he explains.
Companies had a great analytical tool, but they didn’t necessarily have more time or staff to use it. In 2018, companies began to look for a better way to utilize data analysis by shifting to a prescriptive recommendation, where the computer analyzes the insight to provide a recommendation that a person can then act on.
Fullmer expects this trend to continue, even moving toward autonomous action where the computer is also making the decision. “We’ve definitely started to see companies move toward leveraging the big data to allow a machine to make a decision instead—so, leveraging artificial intelligence (AI),” he adds.
The benefits of autonomous decision-making are especially obvious in retail planning, for example, where millions of decisions must be made daily.
“If you’re trying to make tens of millions of decisions a day about what to order, and you add a lot of data, such as what competitors are doing, their pricing and the like, a person just can’t make all of those decisions. They can’t do it at speed,” Fullmer explains.
AI can also aid in executing at scale the service level required in today’s market, which is a direct result of Amazon’s effect on the supply chain.
“Today, the question is how to guarantee the target service level or what we call the service-driven supply chain,” says ToolsGroup CEO Joseph Shamir. “Because of that, there is a need for high scalability and a very high speed of planning that is driving execution.”
AI and machine learning have the potential to drive said execution.
“You can have a huge advantage by leveraging the data that you have in a more sophisticated way. And that is the stage we see a lot of companies trying to move into,” Shamir continues.
Gaps Remain
There are several challenges companies must address, however, before they reach this level of efficiency. The quality and amount of data harnessed is No. 1.
“AI and machine learning, in particular, require a very large amount of data in order to create a model that has a clear signal. These big data are not that available yet, and some of them are not very clean either. They’re in preparation,” Shamir says.
While large corporations like Amazon or Google already have direct access to a very large amount of data on customer or consumer behavior, he adds that “most companies are still working with small data or medium data—somewhere in between.”
Fullmer agrees that the more information or data you have and the higher quality it is, the better the results. But beyond supporting AI, there are still opportunities for companies with limited amounts of data.
“There are companies that would say, ‘I don’t have all of this yet, and so I’ll wait,’ but I encourage them to give it a try,” Fullmer says. “You can do a small-scale project to understand what might be possible, and a lot of companies are surprised at the ability to get some value while you continue to add data.”
At JDA, Fullmer says they are aware that in the real world, data is going to be incomplete, and it’s not going to be completely clean. “We’ve seen some real surprises in terms of what you can do even with limited data…so we’ve focused a good bit of our time on building machines and machine learning that account for the real-world quality of data,” he adds.
Siloed data, and in return, managing it so it’s usable is also a significant problem for most organizations.
“Companies are still trying to understand the enterprise approach to collecting data. We’re still seeing that there are a lot of silos that exist in functions that are starting to collect data. They’ve come up with a place that they’re going to put that data, but that results in a lot of redundancy,” Fullmer says.
He adds that companies need to create a holistic strategy for where their data should reside, and how they then make sense of it.
Use of public cloud providers is one possible solution. In 2018, trust in the cloud increased dramatically as companies began to initiate projects with these providers to create data lakes, which can store data at scale.
“There are some signs of promise there, but how to keep the enterprise data and harmonize that across all the functions is an area that I think we continue to see steeped in challenge,” notes Fullmer.
In regards to data management, Shamir doesn’t see storage technology as the problem, but the need for a new business culture.
“We need to introduce a new business culture not only to enable data collection, but also to manage it in a way that it can be usable by new and powerful technologies,” he says.
What that culture looks like is still to be determined, but Shamir suggests companies start by creating an organizational structure that is not necessarily under the IT department but a chief information officer.
“You need a special unit that is dedicated to data management and data scientists that are working to correctly manage and store the data based on how it can and should be processed in the future,” he explains.