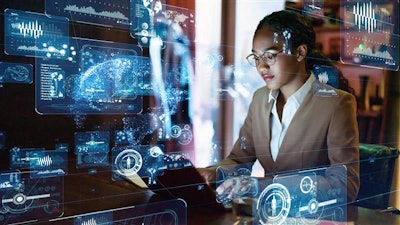
The COVID-19 pandemic has created – or at least, called attention to – many pain points within the supply chain. The past two years have shined a spotlight on just how vulnerable the global supply chain can be to unknown events – things that can be difficult to predict because they simply haven’t been experienced before.
Transportation and logistics companies at every level of the supply chain have faced some of the most unpredictable events we’ve seen in recent history over the past several years, including heightened health risks, extreme weather events, unprecedented shipping delays, labor and product shortages and much more. Even just one of these events has a ripple effect throughout the supply chain, so finding ways to predict the unpredictable is critical to keep things moving smoothly.
That’s where machine learning comes in. With the ability to run multiple scenarios through a variety of different parameters and backed by the power to process immense volumes of data, machine learning algorithms can do much of the heavy lifting that supply chain stakeholders have historically done manually in order to predict the unpredictable and optimize operations.
Because these algorithms “think” differently than humans and can take so many more data points into account, they often uncover creative new insights and opportunities that humans may not have considered. Here are a few ways machine learning can help supply chain organizations unlock creative new solutions:
Freeing up time
Machine learning algorithms benefit from ingesting as much data as possible – something that is not always true for humans, who can only process a limited amount of information before we become overwhelmed.
There are two main types of machine learning algorithms: attended and unattended. In an attended model, a human will guide the algorithm – at least to start – by using their knowledge and expertise to “train” or “teach” it what successful outcomes are (an unattended algorithm would learn on its own).
Once these attended models are trained appropriately, they can soon free up staff time to think more creatively, tackle more strategic work, and/or handle rare exceptions – leaving the manual processes to the algorithms.
And because the algorithms have “learned” from the experts, those valuable human insights are not lost – instead, they are captured and put to work through the machine learning solutions, which are taking into account the human element, but backing it up with data.
A great example of this approach in action is optimized dispatch solutions. Dispatchers famously have a great deal of expertise in their area and are often tasked with figuring out how to assign loads to drivers under many different, complex parameters (such as the type of freight, when to bring them home, available Hours of Service, conditions they’re accustomed to driving in, reducing empty miles, and much more). By training an algorithm to evaluate these many data points while taking those parameters into account, dispatchers can reduce the amount of manual work they need to do to match loads, letting the solution figure out and bring forward recommended assignments. Instead of spending all that time trying to figure it out themselves, dispatchers can review the recommendations and make the final call according to their best judgment.
In turn, this frees up dispatchers to spend more of their time on more meaningful activities like checking in with drivers, managing exceptions and more.
Innovative new insights
While freeing up staff time to be more strategic is certainly a benefit, undoubtedly one of the greatest advantages of using machine learning solutions is the new, creative insights they can unearth. Algorithms aren’t subject to the same “emotion” or personal bias that often factor into human decision-making, so the insights they bring to the table may unlock entirely new ways of thinking.
It’s not uncommon for shippers and carriers to become locked into a mindset of “this is how we’ve done things in the past,” so bringing in a fresh, unbiased perspective – like that provided by a machine learning algorithm – can lead to innovative and creative solutions that may not have previously been considered.
Machine learning algorithms are very helpful in identifying pain points and finding solutions – maybe you need to start ramping up manufacturing more shipping containers. Or could additional drayage carriers alleviate port congestion issues? Why did the grocery store run out of milk – was it because production wasn’t high enough, or were there not enough trucks available to move it from Point A to Point B in time? These are the questions that machine learning can bring a new perspective to.
For example, a machine learning algorithm could help a carrier realize that they need to expand one of their regions by a couple of miles, in order to encompass a town where several of their drivers live—which could mean that those drivers could then operate more efficiently in that region, or make it home more frequently, etc.
Or, an algorithm could identify a solution for regional shippers to find new long-haul opportunities – maybe one driver could bring a load to a “hub” location where it is picked up and taken to its final destination by another driver. And then, the original driver could take another load back from the hub to their home location, meaning they can have more regular working hours, leading to higher job satisfaction and retention.
A power-to-load optimization solution I’ve worked with that’s based on machine learning algorithms has reduced deadhead miles for its users by 2 percent – which as many know is a very powerful number when it comes to increasing utilization. It has also led to a 1 percent improvement of on-time service amongst its users, which is a very promising number in the full truckload/expedited space where delays are common.
Final thoughts
The supply chain relies on predictable and reliable conditions to run at peak capacity – something that realistically often does not happen. However, if even a few of these less frequent but high-impact circumstances could be predicted in advance and provide alternatives, operations would have time to adjust accordingly to keep the supply chain moving.
Machine learning algorithms are more likely to pick up very small details from data that could lead to insights that have huge results, even from making tiny tweaks. And because you’re using human experience and expertise to train the models, you’re not losing out on that “human touch,” either.
By applying machine learning algorithms and tools, supply chain stakeholders can gain a better understanding of how the historical events they’ve experienced in the past can provide insights for the future – the areas of opportunity, creative new ideas to consider, and more. Forward-thinking supply chain organizations of all sizes can benefit from machine learning solutions, which are optimizing operations and keeping the supply chain in motion.