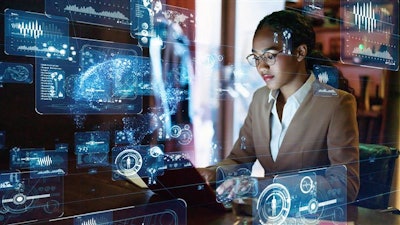
Statistical modeling and mathematical optimization play a central role in supply chain management because of the large number of micro-decisions that need to be made and the necessity to plan operations in advance in the face of uncertainty. Here are several trends that should be on the radar of companies that develop in-house supply chain optimization models or make industrial supply chain management software.
1. New data and methods for COVID-19 and post-COVID-19 times
The Coronavirus disease (COVID-19) pandemic has been the biggest disruptor of supply chain operations since the 1940s. In 2020, managers of supply chains and inventories had to work through numerous shocks related to lockdowns, reopenings, shifts in consumer behavior and economic downturns. These efforts were widely supported by analytics tools and data science teams that adjusted forecasting and optimization models to account for unprecedented shocks. Many of these adjustments were implemented using new signals and data sources not normally used before the pandemic such as seasonal influenza statistics, international data, macroeconomic data for the major crises of 2000 and 2008, and so on. These developments will continue in 2021, and many of the techniques and improvements adopted during the pandemic are likely to be around for a long time.
2. Integrated decision support tools with predictive components
Although decision support tools and predictive models have been widely adopted in supply chain management, it is often a challenge to develop integrated solutions for complex chains that have multiple types of warehouses (e.g., for regular, bulky or perishable products) and multiple distribution channels and service level agreements (e.g., regular or same-day delivery). Currently, many companies are in the process of developing comprehensive service lineups that enable them to efficiently search for the optimal supplier and logistics options while accounting for these complexities, and we expect this trend to continue in 2021 and beyond.
3. Adoption of prescriptive methods
Demand forecasting has been widely adopted in the industry, and it is a foundation for many supply chain planning and optimization activities, but many companies have not yet managed to develop comprehensive decision automation capabilities on top of such forecasting. Manual analysis of forecasts and other predictive outputs will be replaced by systems that have a higher level of automation and combine statistical, econometric and risk scoring models to make decisions more autonomously. More specifically, simulations and reinforcement learning will be important technical enablers of this process.
4. Adoption of simulation and reinforcement learning methods
The development of both integrated solutions for complex supply chains and better decision automation requires models and mathematical methods capable of evaluating complex multi-step scenarios while accounting for uncertainty and stochastic patterns. In such settings, it is quite challenging to use traditional optimization methods because the complexity of model development and maintenance can be prohibitively high. On the other hand, simulation-based methods and advancements in reinforcement learning enable optimization using simpler procedural models of the supply chain environment that unlock new levels of decision automation. However, this approach has its own challenges because the number of decision variables and entities that participate in the process are typically extremely high. Nevertheless, these technologies to evolve and play an important role in 2021 and beyond.
5. Omnichannel scenarios will be first-class citizens
Over the last few years, many B2C companies have adopted omnichannel capabilities such buy-online-pickup-in-store (BOPIS), and many B2B businesses have either developed their own e-commerce platforms or integrated with marketplaces. These developments have elevated the role of omnichannel inventory management and introduced additional challenges and complexities. For example, BOPIS functionality requires a retailer to manage in-store inventory reservations in a way that maximizes the online exposure of the inventory while minimizing the number of fulfillment exceptions caused by out-of-stock situations. This scenario requires new types of predictive and optimization models, and so do other new omnichannel use cases. The adoption of cross-channel features and capabilities will continue in 2021 (and will even accelerate after the end of the pandemic), and this will drive the development of the corresponding feature in supply chain management and optimization software.