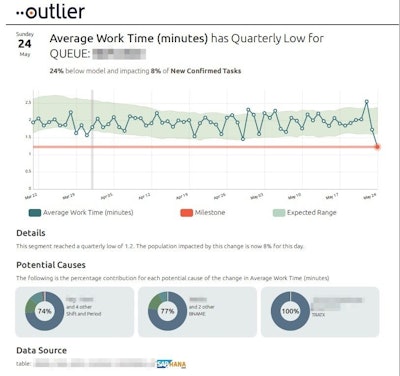
During a recent conversation with a large consumer packaged goods (CPG) brand, the topic of using artificial intelligence (AI) to improve supply chain visibility came up. The team was looking at multiple data sets from their supply chain, but were unable to connect the dots between the multitudes of alerts and the inventory disruptions they were experiencing. They needed a cohesive view of the supply chain to quickly pinpoint problem areas and take action to improve customer experience. Business intelligence (BI) dashboards only gave them part of the story, but were not elevating clear answers. And, despite understanding the potential value of AI in increasing the scope and speed of analysis, they had no idea where to start.
In high-volume, high-velocity environments like CPG manufacturing, there are a lot of data captured in multiple systems every hour. Not only does it take an enormous amount of time and staff to analyze, but any insights garnered from the data have a greatly reduced impact by the time they are extracted, surfaced and acted upon. The key to identifying areas of disruption in the supply chain that can be addressed quickly – in order to mitigate risk or take advantage of a positive trend – is to know what’s happening in your data immediately.
Real-world use cases
To create this immediate and actionable visibility, a few things need to happen. First is connectivity – creating a way to connect all the data together. Second is constantly querying the data. And third is using tools like AI to automatically elevate the right insights from the continuous queries that isolate the exact information you need. When done right, this can lead to significant changes in a business by providing organizational access to insights that would have previously gone unnoticed.
BI is one part of this equation. But an automated business analysis (ABA) approach on top of BI is an essential new component that tells supply chain managers what they most need to investigate at any given time. ABA quickly identifies the unexpected changes in data that matter most, acting as a virtual business analyst, constantly combing through all available data, looking for the most impactful developments.
Across stages of the supply chain, ABA serves different purposes. But, it can be viewed as an umbrella analytics engine for the entire organization. Here are some use case examples of how ABA uncovers critical and timely insights across different areas of the supply chain.
Demand planning. A key aspect of demand planning is to identify meaningful changes that emerge between forecast and actuals to reduce the gap. This requires insight into when and where materials or products are needed, and what internal or external market forces might disrupt the current delivery plan. ABA can create connectivity across the sales and operations planning function and demand planning function, enabling teams to understand real-time changes in supply, demand and customer behaviors, and take immediate action to ensure that productivity and delivery are minimally impacted. By uncovering the root causes of repeat disruptions, demand planners can create new approaches, options or increase flexibility among standard processes so future disruptions are minimized.
Manufacturing. To optimize manufacturing, operations leaders need to understand key trends, triggers or alarms and unexpected changes in predetermined KPIs across the manufacturing process. ABA serves as the connection between fragmented manufacturing data sets to deliver a complete view of where disruptions occur along the entire process. By addressing these issues quickly, organizations can boost yield and improve efficiency across the entire supply chain. Insights from an ABA system can be immediately shared with leadership teams all the way down to line managers to ensure fast remediation.
Fulfillment. When it comes to fulfilling demand, operations and fulfillment managers require transparency across both inputs and outputs. ABA can sit across all relevant data sets to alert managers about changes, deliver transparency and identify opportunities to improve on core KPIs. It simplifies a very complex fulfillment environment, enabling managers to make decisions that improve customer service levels while reducing the overall cost of delivery.
Transportation and logistics. Transportation and logistics represent a very high cost for organizations, so issues here can have significant financial impact. Reducing waste, incomplete and late deliveries at this stage are critical metrics. ABA can flag missed metrics immediately so organizations can modify production and logistics schedules, reduce errors, reshuffle routes or deliveries and avoid penalties to minimize unnecessary costs.
Warehouse operations. Warehouse operations are often a starting place for organizations looking to increase task efficiency or reduce latency. By identifying meaningful trends and changes in warehouse operational and efficiency data, companies can reduce another big expense. Breaking down meaningful changes in core KPIs across days, shifts or even by the hour, creates opportunities for process changes and personnel training that can create permanent incremental warehouse operational improvements.
Exponential benefits from uncovering the unknown
For most companies, adding a layer of AI to identify unexpected changes in data adds exponential value to an organization’s existing software investments. While standard BI dashboards offer a repeated snapshot of commonly used, top-level metrics, AI captures areas of opportunity that would otherwise be missed, leading to operational improvements, a better employee and customer experience, and stronger financial performance. ABA sees what managers are not looking at — or looking for.
For example, one CPG company was managing warehouses with hundreds of employees receiving and shipping perishable food products. Using ABA, they discovered a quarterly low metric for the amount of time it was taking to start and complete a task in a particular warehouse queue. The average work time for this stage was significantly shorter than average. Leaders wanted to figure out how to replicate this improved process across other queues to create a more efficient workflow for their entire operation.
By quickly integrating SAP HANA data into its ABA tool, the CPG brand identified positive queue activity among specific workers. The company then identified what these workers were doing differently and used these learnings and practices to optimize other queues within the warehouse, recognize productive workers, and drive overall output and sales. Without ABA, this unexpected improvement would never have been discovered, missing an opportunity to improve overall operations.
A supply chain that can rapidly evolve with changes in the market while constantly improving its own internal operations is a distinct competitive advantage. By seeing subtle changes in the mountains of data collected, leaders can act quickly and take advantage of them long before competitors catch on. The use of AI-enabled technologies like ABA is growing quickly and the tools are easy to apply to existing data sets, helping organizations provide a better experience and quickly capture more revenue by simply following the insights in their own data.