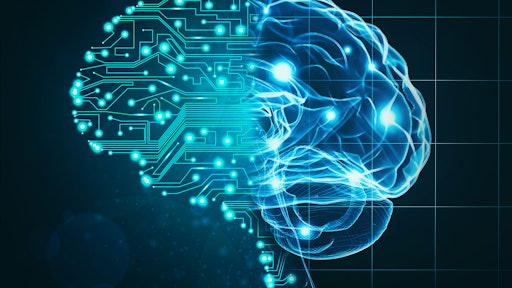
In today’s technology-driven world, many organizations still view artificial intelligence (AI) with an element of fear and uncertainty. As a result, the tangible benefits that it brings to sourcing and procurement are still largely untapped. But, buried within the wealth of raw information that exists in both internal and external systems are critical strategic, customer and operational insights.
AI can help illuminate the insights hidden in this data, enabling sourcing and procurement teams to validate or clarify their assumptions, inform decisions, and chart new paths into the future.
Here are six potential use cases of AI in sourcing and procurement.
1. Spend classification and enrichment
There is an increasing need to better understand internal spend (the three Ws: who spent, on what and with whom), along with a need for more robust processes to manage compliance with external contracts and internal policies. Accurate spend data is foundational in the development of effective category, sourcing, and spend management strategies. Spend classification technologies are now allowing even better analysis and, in some instances, enriching this data with external information.
Projects that use AI spend classification have typically been able to achieve a very high level of accuracy in the classification of data. This has led to increased precision in the analysis of spend information, driving more value for an organization.
2. Global sourcing insights
Chief procurement officers (CPOs) often run into a maze of data when looking to determine their sourcing strategies. New tools are now enabling businesses to use the power of AI to explore high-level sourcing strategies. These enable CPOs to identify where to source specific products and evaluate the savings potential against corresponding risk.
Organizations are able to critically evaluate sourcing opportunities through data driven product and supplier insights, allowing them to better understand key industry and macroeconomic trends. This enables businesses to create transparency in the supply chain, identify new sources of supply and shift category spend to a more optimal allocation.
3. Invoice data extraction
One of the more established uses of AI in accounts payable teams today is in the extraction of invoice data. These systems generally consist of a data extractor with an AI component for automated extraction, a document manager controlling the workflow and a verification interface for operator-based data capture. For those who don’t have source-to-pay systems with e-invoicing in place, this can be a valuable solution, and it’s fast to implement.
The implementation of invoice data extraction can reduce both manual processing times and fraud. In instances where spend data has not been captured at a granular level of detail, these tools can also be used to process historic invoices to provide more insight into an organization’s spend patterns.
4. Automated compliance monitoring
Where organizations haven’t adopted a source-to-pay system, compliance can be a manual, time-consuming process. Even for organizations that have embraced these solutions, there are likely to be a large number of suppliers that are not enabled within the system. For these situations, AI can be used to help structure the contract, invoice and PO data to identify and highlight non-compliance.
Organizations that adopt these solutions can see a reduction in spend due to fraudulent or erroneous transactions and a reduction in processing times for the auditing of transactions. By helping clients limit leakage and preventing inaccurate payments across third parties, they are able to capture maximum value from their contracts.
5. Contract data extraction
Using a similar technique to invoice data extraction, contract data extraction allows an organization to structure large volumes of unstructured contract data. At a basic level, this allows organizations to upload large volumes of contracts that may be in PDF, image or Word file format and then extract key information such as start dates, end dates, payment terms and references to master agreements.
For procurement departments without structured contract databases, using natural language processing (NLP) to extract key information and terms can help in compliance and accelerate the transition to systems. In addition, advanced solutions can help determine the risks within a contract, highlighting specific areas for review.
6. Contract lifecycle management
Despite the fact that contract lifecycle management (CLM) has been around for decades, AI is now beginning to make an impact in this area. CLM tools that use AI have the ability to support contract generation, contract negotiation, and the identification of risks within contract language.
Organizations using an AI-powered CLM system are able to significantly reduce the time required to generate and negotiate with counterparties, as well as more easily identify discrepancies in contracts. In the context of procurement teams, these technologies are also able to support and facilitate large change projects such as mergers and acquisitions; specifically, the identification of assignment or termination rights and the negotiation of corresponding consents.
AI is here now and is already having a profound impact on how we live, work and do business. Companies, governments and organizations must embrace innovation in order to reach the places inaccessible through current technologies and processes. AI can and will transform organizational decision-making and drive efficiencies. However, like all revolutions in technology, capturing maximum value and minimizing risk will require an in-depth understanding of what AI is and how it aligns with your core business. Recognizing the growing importance of AI is the first step. Organizations must then understand how to move from theory to meaningful business implementation if they are to create a sustainable AI transformation.