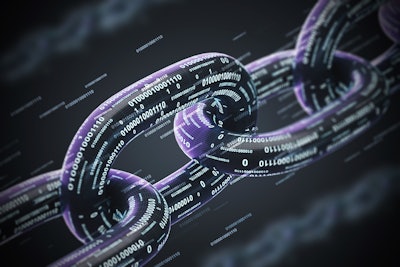
The potential for artificial intelligence (AI) to improve supply chain processes has been an area of focus for supply chain-oriented companies for at least 5 years now, but that attention has increased following the disruptions caused by the Coronavirus disease (COVID-19).
COVID-19 has placed a magnifying glass over supply chains, as manufacturers, retailers, distributors and consumers experienced delayed deliveries as a result of market closures and quarantine mandates. AI holds the potential to automate certain supply chain processes and improve overall supply chain management, reducing the potential for similar supply chain upsets in the future.
Indeed, a majority of supply chain leaders are prioritizing using automation to perform routine tasks and 60% expect to be taking advantage of AI-powered capabilities within five years. However, only 12% of current supply chain professionals said their organizations were currently using AI in supply chain operations. So, how can supply chain-oriented businesses get ahead of the curve when it comes to AI adoption, and leverage the critical benefits provided by AI before their competitors do?
AI for the supply chain
First, let’s review the benefits of AI for the supply chain and why it’s such a high priority for supply chain-oriented businesses.
AI is receiving a lot of attention from supply chain leaders for a multitude of critical benefits that the technology can provide to supply chain-oriented companies. In fact, supply chain management is one of the business use cases for AI that is expected to generate the highest ROI for early adopters and high performers. Specifically, AI has been recognized as a major revenue driver for supply chain organizations when designed for demand forecasting, logistics network optimization and spend analytics.
AI for distribution centers offers some of the most attractive cost-reducing capabilities to distribution center owners and managers. The concept of a “lights-out,” fully automated distribution warehouse has been around since the 1980s, but recent AI and technology developments are making that goal more achievable for supply chain-oriented businesses than ever before.
Logistics companies also stand to reap huge benefits from AI. As AI-driven cars become more common and the AI technology that pilots them becomes more advanced, logistics companies around the globe will have the opportunity to fully automate their delivery operations, reducing the risk of delivery delays and errors.
In addition, AI and machine learning (ML) can help logistics companies to optimize shipping operations to ensure that the most efficient delivery routes, times, and methods are used. Logistics companies can feed data surrounding weather reports, customer feedback and historical delivery information into AI programs to accurately predict future delivery times. AI-supported supply chains can improve their operational efficiency to become up to 45% more effective at on-time delivery with fewer errors.
With all of these benefits for supply chain organizations, it’s easy to see why AI is such a huge priority for supply chain managers and leaders. However, if so many supply chain-oriented companies are going to be developing, training and deploying AI solutions in the next five years, they will need to rapidly implement a robust, consistent and cost-effective source of supply chain data to fuel their AI if they hope to beat their competitors to the final destination: an AI-enabled supply chain.
Leveraging improved integrations to fuel AI
AI programs are fueled by data. The accuracy, scope and capabilities of an AI program depend entirely on the data it is fed to learn how to execute the task for which it was designed. However, that data must be organized and labeled in a machine-readable format before an AI program can digest it. If an organization uses EDI or PDF as a means of exchanging information between partners and suppliers, that information is not fit for machine-readability in its current format.
Modern integration technology can compile data from previous interactions with a business’ ecosystem of suppliers, partners, traders and customers in a format that is structured to be consumed by machines and AI programs. A well-built integration platform will also connect legacy, on-premise systems that might use paper, PDF or forms of communication such as EDI to cloud-based applications that use API. This enables organizations to take full advantage of the data they have accumulated over the years that might be stored in legacy systems, without sacrificing any end-capabilities of their AI initiative.
This machine-readable data can then be fed directly into an AI program that would learn from previous interactions and be trained to make predictions for the future based on those interactions. Therefore, AI programs become exponentially more robust and accurate as they are fed exponentially higher amounts of quality data.
While training an AI program to predict “black swan” events such as the COVID-19 pandemic would require an amount of global data unattainable by the vast majority of supply chain-oriented businesses, organizations can leverage AI to identify and flag situations that could lead to supply chain upsets. This enables supply chain businesses to plan ahead and prepare contingency plans for any delivery delays or distribution upsets that may come their way.
Further, if an organization notices that their AI programs are red-flagging multiple situations that could impact supply chain processes at once, they can invest additional human and machine resources into investigating the issues and potentially identifying an upcoming black swan event before their competitors. Therefore, fueling AI programs with data from ecosystem integrations is critical to the success of supply chain AI. If an organization is training their AI program with every available piece of data surrounding their ecosystem of suppliers, partners, traders and customers, there is much greater potential to identify even the smallest clues that can uncover critical insights into the future of their supply chain operations.
Many supply chain-oriented businesses are scrambling to deploy AI programs that support the critical capabilities they desperately need right now. However, even the most robust AI is rendered useless without adequate, machine-readable data to fuel AI insights. Ecosystem integration is a resource-effective means of not only compiling data for AI program training, but also ensuring that data is presented to the program in a machine-readable format, ultimately enabling supply chain organizations to train and deploy AI programs on a faster timeline and with more accuracy than their competitors who do not leverage modern integration technology.