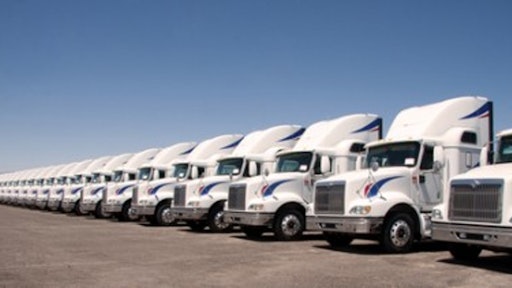
One of the most challenging aspects of the supply chain is the ability to look ahead and forecast supply, demand and future transportation needs. The days of making decisions based purely on historical patterns and instincts are well into the past. Data and analytics are now deeply entrenched into the planning and execution phases of any supply chain, and the challenges with the Coronavirus disease (COVID-19) has made this even more critical. Even though the use of analytics has only emerged over the last 10 years, the ability to leverage this data to forecast or predict jobs and outcomes based on several factors is quickly evolving as the Next Big Thing for data use. Today’s transportation fleets are leveraging this advanced business intelligence to help drive costs out of the supply chain.
Using historical patterns to forecast future decisions
Predictive data leverages science and analytical trends to create algorithms and formulas that combine economic insights along with data mining trends to arrive at a forecasted output that is scrutinized for more accurate logistics planning.
When making critical business decisions, predictive modeling can be extremely beneficial in helping fleet executives leverage past and present data. These organizations have relied on data to leverage key insights to help determine Fleet Advantage’s TIPPINGPOINT, the point at which it makes more financial sense to replace an aging truck in the fleet with a newer, more efficient and safer model.
However, today, tech-savvy organizations are using predictive modeling to help forecast this TIPPINGPOINT by looking into the future and selecting specific dates to gain visibility into the future lifecycle performance and replacement economics of each truck. Fleets can analyze any number of criteria, including truck make, model and type, as well as utilization characteristics such as usage location and fuel metrics. This critical insight helps predict the savings over the next one, two, or three years and shows where unit replacements would present operational cost savings over the predicted time allotment during that time span.
Where companies are leveraging data for optimization during COVID-19
The closing of restaurants and increased demand for grocery deliveries means that supply chain routes and typical business patterns have shifted for many trucks and drivers. As a result, transportation fleets must leverage truck utilization data daily as well, load percent capacity, driver wellness and other KPIs to monitor the efficiency and optimization of each unit. While it’s important to take an “all-hands-on-deck” approach to help deliver goods and items promptly, fleets need to keep an eye on truck utilization to maintain the right life-cycle management of each unit in the long term.
Additionally, it is essential to have advanced data feed capabilities that upload in real-time compared with backward-looking data that only looks at the previous month’s data. This is crucial for managing through items such as mileage, miles per gallon, idle time, routing and driver hours, and will allow fleets to most efficiently navigate through the extraordinary pace of changes due to COVID-19.
How data forecasting keeps budgets in line
Aside from the new challenges from COVID-19, the ability to arrive at more accurate business outcomes helps create more targeted budget scenarios. Legacy systems and approaches, while they relied heavily on historical data patterns, forced executives to make educated guesswork out of forecasting. When you combine this inability to accurately forecast projects along with the unpredictable market and supply-side prices, the recipe for budgeting disaster is illuminated and very real.
Predictive modeling allows organizations with transportation fleets to create future business insights with a significant degree of accuracy. With the help of sophisticated data analytics tools and modeling, these firms can now use past and current project utilization data to reliably forecast budget trends milliseconds, days, or years into the future.
Predictive modeling and analytics tools are expected to reach approximately $10.95 billion by 2022, growing at a compound annual growth rate (CAGR) of around 21% through 2022, according to Zion Market Research.
Why predictive data increases decision accuracy
There are a few reasons why today’s predictive modeling data is more accurate than what organizations had access to in years past. Predictive modeling is primarily based on actual, empirical data and macroeconomic insights from historical outputs and present-day models. It is far more elaborate than the forecasts based on the theory used in legacy business operations.
Empirical data is based on “evidence” derived from previous cost data and other criteria that have proven themselves in actual real-world scenarios. These data outputs are then formulated into precision-based models and scenarios that offer visibility into accurate forecasting techniques organizations and data scientists today use to arrive at certain economic conclusions in their decision making as explained in the budgeting scenario above.
Data and analytics have enabled organizations to streamline their businesses and create efficiencies never before realized. What’s more is, this advanced level of business intelligence helps organizations navigate through unprecedented circumstances such as what is being felt with COVID-19. Companies with transportation fleets that leverage predictive modeling technology will increase their bottom-line efficiencies even further and amplify their competitive advantage post-pandemic.