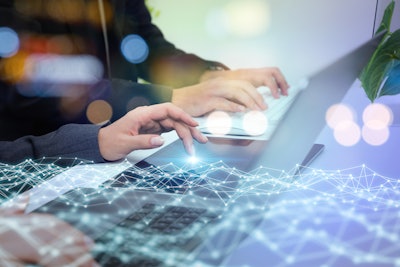
As more manufacturers incorporate artificial intelligence (AI), machine learning (ML), natural language processing (NLP), and other technologies into the workplace, they’re essentially replacing manual work with automated processes. This helps free up human resources to focus on more important things while also enhancing operational efficiency and productivity across the organization.
For engineers, advanced technologies like AI, ML, NLP, and digital threading can help to vastly improve the knowledge management process, much of which is still handled either manually or by using disparate data systems that don’t “link up” to one another. With organizations wasting up to 30 percent or more of their engineering and R&D resources duplicating work or repeating past mistakes, finding and accessing information stored in those repositories—often scattered across an organization—presents unique challenges.
In an increasingly complex manufacturing environment, where information from past projects is often buried in decades-old, non-integrated enterprise systems, engineers can spend 40 percent or more of their time searching for information. The scenario is only growing in complexity for firms: Gartner predicts that the volume of data being generated will grow by 800 percent over the next four years, with 80 percent of that information being categorized as unstructured data.
In this article, we explore the manufacturing environment and show how advanced technologies like AI, ML, and NLP are helping companies work smarter, better and faster without having to add human labor or make significant changes to their current R&D processes. We’ll look at what’s driving adoption of these technologies by engineers, scientists, and other knowledge workers, and provide a few starting points for companies that want to get started with AI, ML, NLP and digital threading.
What Are These Advanced Technologies?
As with any new innovation or concept, the definitions of AI, ML, NLP and digital threading change depending on whom you’re talking to. Here’s how we define them at IHS Markit:
Artificial Intelligence
One definition of intelligence is the ability to process information and then apply the knowledge and learn from that information. Going a step further, AI or computer intelligence is the ultimate ability of a computer to process information—be it data, visual information, auditory information, written information—and then use it to solve problems in the world today.
Machine Learning
Focused on how a computer takes information in and parses it to discover patterns of interest, ML is important because a computer is only as “intelligent” as the data set that it’s given. Put simply, you have to train a computer to know what the Mona Lisa is if you’re writing an algorithm that’s meant to identify this famous subject in a child’s drawing. You can collect a lot of drawings of the Mona Lisa created by children around the world and then expose those to a ML algorithm (which would then take those examples and learn to produce a program to best depict the Mona Lisa for the program).
Natural Language Processing
A particular type of artificial intelligence, NLP focuses on teaching a computer to understand the written word—or basically, training the computer how to read and understand what we write today. This is especially relevant for engineers who have to sift through large volumes of unstructured data to get the answers that they need. Whether it’s research, design or manufacturing, every single aspect of product development today involves the written word. A technician working on a product on the floor needs to perform a certain operation in manufacturing, but does he or she have time to read a 100-page document to understand how to do that operation correctly? Probably not. That’s where NLP comes in and makes the culling down of that information very streamlined, automated and easy.
Digital Threading
The digital threading concept utilizes all of these technologies to significantly raise the stakes on sifting through big data to connect critical information. Consider a physical asset: a person, a product, a part number. The goal of digital threading is to create a connected flow of information about the asset, and all data related to the asset throughout its life cycle. Taking the example of the asset being an automobile, the idea is to connect all design, manufacturing and performance data throughout the automobile’s life cycle. Traditional enterprise software systems can identify explicitly linked information, such as a document number written and referenced in another document. But the real power comes in uncovering implicit links; creating navigable relationships that don’t currently exist outside of an engineer’s mind. A simple example of an implicit link would be connecting an expert in subject matter to a very specific written requirement. Establishing implicit links requires really powerful AI. Now, imagine the power of having this information in an enterprise 5,000 engineers strong. We are talking about delivering the right information at the right time in an astoundingly complicated environment.
The Devil is in the Data
Ever since the invention of the computer in the 1930s, engineers and scientists have been using computers to solve problems like humans did in product development, product life cycle and manufacturing. As a whole, society has done an astounding job of using computers and applying computer calculations to solving problems in automation and digitization of product research, design and manufacturing.
Along the way, we’ve created a ton of big data—a proliferation that’s only been multiplied in the cloud computing era. The problem is that we only use about 10 percent to 20 percent of this big data, and the rest is just sitting dormant in disparate, siloed repositories. The question is, how can we use advanced technologies like AI, ML and NLP to teach computers how to learn from this information and process it at a much faster rate than the human brain can?
Manufacturers are particularly well positioned to take advantage of these advanced technologies. Consider the product lifecycle for a mobile phone. From R&D to manufacturing to ongoing support for that product, the data that’s being generated is literally stored all over the place. It’s also in different formats, be it structured, unstructured, numerical, text and so forth.
For most product companies, tracking all of the data and then transforming it into useful intelligence is 100 percent human-reliant. Engineers struggle under the pressure of having to locate that information, process it, learn from it, and then apply it to ensure a good product outcome. The struggle is pretty much an accepted nature of the business, and it’s why AI and other technologies are becoming more and more attractive for manufacturers right now.
But while the AI buzzword is everywhere, we’re seeing a major disconnect between projected AI market size and the actual organizational implementations (less than 20 percent, by our accounts).
The fact is, people have a feeling that computer intelligence can be used to process data and learn from it, but we’re still struggling with the basics of actually implementing AI, ML, NLP and other technologies in product development.
Asking the Tough Questions
Making technical products today requires millions of different parts, all of which are needed to build out the final products. That means engineers and scientists around the world are creating bills of materials and then reverting to those lists to locate single part numbers (e.g., in order to find the item’s design specifications). As part of this process, the following questions probably come up:
- Is this part number included in other product designs and bill of materials?
- What’s the performance data of this part?
- How do I know what suppliers I can order it from?
- What are those suppliers’ written specifications?
- Is this product mentioned in the Lessons Learned reports?
- Have we ever had problems in product use with this specific part number?
- Are there any alternates to this part number?
If you’re an engineer who is looking for answers to all of these questions, you’re going to spend hundreds (if not thousands) of hours finding that data and answering those queries. You can research and read and make connections across the data you’re gathering, but you’ll also miss key pieces of information along the way. It’s not a matter of “if” you’ll miss that information, but rather, just how much information will be missed.
Using digital threading, engineers can close those gaps and then leverage AI, ML and NLP to read the documents for them and establish implicit links. With the concepts threaded together, the tribal knowledge collected, and the research process automated, engineers can move forward instantaneously with risk of error removed at all steps of the product development process. You simply can’t ignore the power of AI in this scenario.
The Key: Focus
With AI, ML and NLP gaining ground in the manufacturing world, now is the time to start exploring just how these advanced technologies can help your organization make better use of its knowledge workers while also eking more value out of the data that it generates on a daily basis.
The key to success is to focus. If you try to start big, and if you try to implement AI across the organization without understanding your use cases, you’ll only be setting yourself up for failure.
Think of how a human is trained; we don’t jump to our Ph.D. thesis when we’re young. We start small. It’s the same with AI and other technologies. Know your use cases and then start with a focused AI-enabled solution and the technology that will solve your acute problems and really provide measurable results.